AI in Business Intelligence: Cost Considerations & Budgeting for Success
- SoftudeFebruary 28, 2025
- Last Modified onFebruary 28, 2025
With today's rapidly changing business environment, businesses increasingly use data to make decisions. Artificial intelligence is a major driver of turning raw data into useful insights. Business Intelligence (BI) solutions fueled by AI enable businesses to analyze enormous amounts of data in real time. The technologies, however, are not cheap. In this blog, we discuss the cost implications of AI in BI and provide pragmatic budgeting tips for success.

Understanding AI in Business Intelligence
AI in BI is not only about faster data processing. It is about leveraging machine learning and natural language processing to extract trends and predict results. AI helps businesses automate cleaning data, reporting, and even predictive decision-making. The applications save time from manual labor and improve accuracy. For instance, AI dashboards can point out anomalies and predict trends, which allows managers to respond promptly.
Businesses that implement AI in BI generally experience better decision-making. They can find obscure patterns within customers' actions and market trends. These patterns aid in the improvement of supply chains and marketing campaigns. Still, with all these benefits, the expense of implementation and upkeep of AI tools continues to worry.
Breaking Down the Cost of AI Implementation
When planning an AI investment, businesses must consider several cost categories. Each category requires careful evaluation.
Upfront Investments

- Hardware and Infrastructure
AI models require robust computing capabilities. Businesses have to spend money on servers, GPUs, and network hardware. This costly equipment can form the lion's share of the budget. Cloud-based services can provide a cheaper option for smaller businesses. Accessing a cloud platform serves to spread costs over time rather than a one-off payment.
- Software Licenses and Tools
Most AI applications involve proprietary software licenses. Such licenses may cover machine learning frameworks, data analytics products, or BI platforms. The prices in these cases can be quite divergent depending on the vendor and the deployment size.
- Data Integration and Storage
Effective AI in BI relies on quality data. Getting data warehouses ready and making different systems talk seamlessly is necessary. This integration will take time and capital. Upfront expenses can cover database configuration, software to administer data pipelines, and data cleansing and structuring costs.
Operational Costs

- Maintenance Costs
Once the setup is done, businesses have to pay for maintenance and upgrades. AI models require retraining from time to time to continue being effective. Constant hardware and software updates keep the system in top condition. These operational costs accumulate and must be factored into any budgeting plan.
- Energy Consumption
Operating high-power computing equipment requires a lot of energy. Data centers have to spend money on cooling and energy management systems. This is a significant operational cost for businesses that house their own servers.
- Workforce Training
Businesses must train employees to use new AI tools by providing courses and certifications. These training sessions boost productivity, although they add costs. Therefore, businesses must plan for continuous learning to keep staff up to date.
Hidden and Indirect Costs
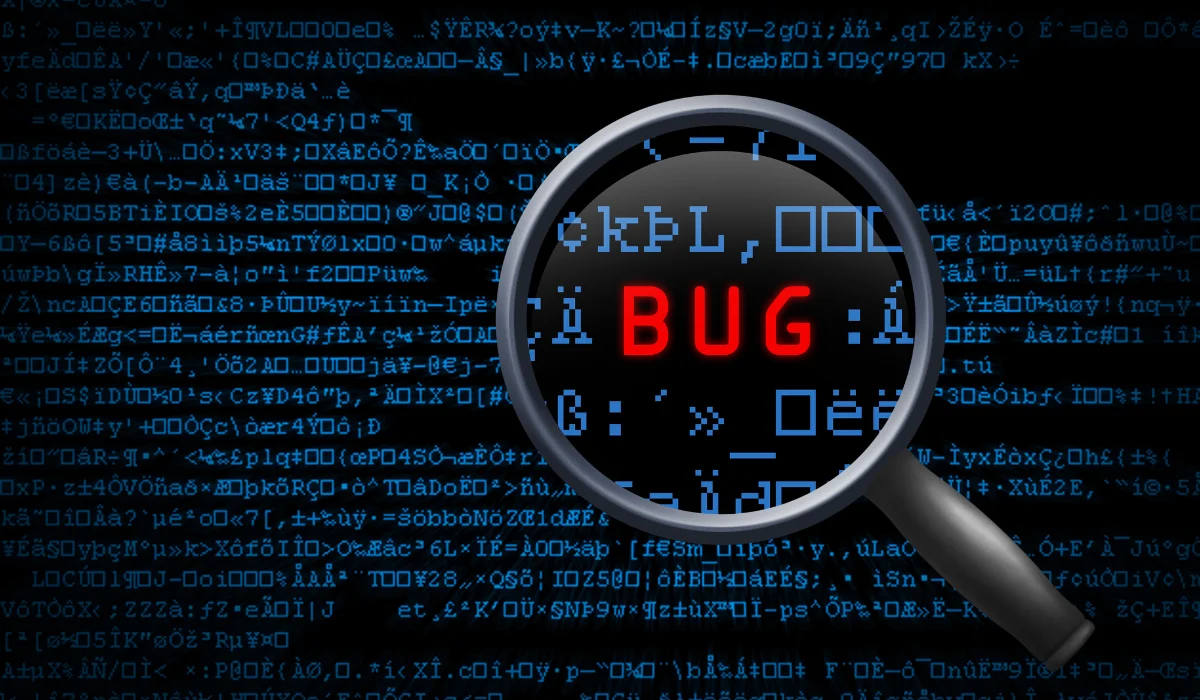
- Customization and Integration
Each business has specific data requirements, which could be costly to personalize AI models. Integration with legacy systems could involve outside consultants. These expenditures are not always immediately apparent but tend to disproportionately affect the overall cost.
- Security and Compliance
Data breaches and regulatory noncompliance can be extremely expensive. Investments in compliance and cybersecurity are, however, essential. AI systems need to be secure and compliant with privacy regulations. Regular audits and security protocol updates contribute to the expense.
- Scalability Challenges
When businesses expand, so do their AI systems. Scaling up may require more hardware, higher-level software licenses, and increased data storage. Growth planning, though the present requirements are humble, is key.
Budgeting for AI in Business Intelligence

Since the costs are high, businesses must remain disciplined in their budgeting practices. One key measure is to perform a rigorous ROI analysis before investing in AI. Firms must determine ROI by defining success such as faster reports, better forecasting, or less manual work, and setting clear, measurable metrics.
Next, businesses should quantify savings from automation and improved decision-making, then weigh these benefits of AI against upfront and ongoing costs. Finally, determine the payback period; while a short period is ideal, long-term gains may justify a slower return.
1. Start with Pilot Projects
Rather than a nationwide rollout, most businesses are aided by a pilot project. A pilot helps a company test the AI system in a controlled environment. This strategy has several benefits:
- Risk Mitigation: Businesses can minimize their exposure if the project fails to deliver as hoped by beginning small.
- Incremental Investment: A pilot involves a smaller upfront investment. After the pilot demonstrates successful outcomes, the budget can be raised incrementally.
- Learning Opportunity: A pilot study provides useful knowledge. Pilot feedback can be used to hone the system before a full release.
2. Leverage Cloud-Based Solutions
Most organizations opt for cloud-based AI solutions to minimize initial costs. Cloud platforms offer scalable computing power on a pay-as-you-go model. This is flexible and minimizes the need for heavy capital investment in hardware.
- Lower Initial Costs: You do not have to make huge investments in physical infrastructure. Pay for what you consume instead.
- Scalability: Cloud platforms are easy to scale. As your requirements increase, you can scale up your usage without much lag. The cloud provider does most of the maintenance work. This minimizes the necessity for a large in-house IT staff.
3. Use Open-Source Tools When Possible
Open-source software has become a strong competitor to proprietary software, particularly in business intelligence and AI. Most are available at minimal or no cost, drastically decreasing the expense of purchasing costly software licenses. Organizations gain substantial cost savings from utilizing these low-cost or no-cost solutions.
Furthermore, open-source systems have powerful communities of developers behind them who continuously exchange knowledge, updates, and best practices to sustain and enhance the software. Furthermore, the solutions provide a high level of customization, enabling businesses to reshape the software to their precise specifications.
While this flexibility is a significant strength, it may mean recruiting skilled staff to put these changes into effect efficiently. In general, open-source software offers a low-cost, community-driven, and flexible platform for organizations seeking to improve their AI and BI capabilities.
4. Negotiate with Vendors
Don't take the initial price when buying AI solutions, bargaining can save you a lot. Large-scale deployments may also be eligible for bulk discounts, so if you intend to expand, use that as leverage.
Certain vendors bundle hardware, software, and support into one service, which reduces costs overall. Also, it is a good idea to seek out flexible contracts that accommodate changes as you change, as this flexibility is particularly valuable in the fast-changing world of AI.
Practical Tips for Budgeting Success

To control the expenses efficiently, businesses need to be proactive. Here are a few helpful tips:
- Plan Ahead: Create a detailed budget with all the possible expenses (hardware, software, maintenance, training, and hidden costs). This will prevent surprises down the road.
- Monitor Spending: Track expenses regularly. Compare spending with the budget and make necessary adjustments.
- Invest in Training: Invest in staff training. Trained employees can maximize AI utilization and minimize the risk of expensive mistakes.
- Review and Revise: The business landscape and technology are constantly evolving. Periodically review your budget and update it to incorporate new trends and challenges.
- Focus on ROI: Always track the effect of AI investments. Utilize precise metrics to measure success and make educated decisions regarding scaling the system.
- Seek Expert Advice: Get advice from business intelligence and AI experts. Their advice can guide you in negotiating improved terms and implementing cost-saving strategies.
Conclusion
In short, AI-powered business intelligence can revolutionize decision-making and increase efficiency. However, success will depend on meticulous budgeting to pay for up-front, operational, and stealth costs. Beginning with pilot projects and using cost-effective tools can create consistent, scalable expansion. Organizations can convert high initial costs into long-term benefits through disciplined planning and ongoing assessment.
Consult with our experts for leveraging AI in your business intelligence workflow.
Liked what you read?
Subscribe to our newsletter