5 Critical Pitfalls to Dodge for Successful AI Integration in Business Intelligence
- SoftudeFebruary 20, 2025
- Last Modified onFebruary 24, 2025
Business Intelligence (BI) has been the cornerstone of data-driven decision-making for a long time. With AI on the scene, BI evolves from static reporting to dynamic forecasting, anomaly detection, and real-time analytics. Integrating AI into business is not a plug-and-play activity, though. It demands strategic planning, data discipline, and alignment of business objectives.

Most businesses, in their haste to integrate AI, hit roadblocks that hinder progress and reduce returns. We have analyzed five key pitfalls businesses fall into when integrating AI into their BI environments and have some practical tips to avoid them.
Integrating AI into Business? Common Pitfalls You Might Encounter
Pitfall #1: Insufficient Clear Objectives

AI is no magic wand that overnight turns BI into gold. It is a sharp tool that needs clear objectives to yield value. Most businesses embrace AI without setting concrete goals, throwing money down the drain with disappointing outcomes. The issue usually lies with soft aspirations like "simplifying insights" or "streamlining analytics" without tangible targets.
Why This Is a Problem
AI models live on clearly defined use cases. Without definition, companies risk deploying AI solutions that create too much data noise with no actionable insights. A sales organization, for example, might require AI to optimize demand forecasting, whereas an operations organization can use AI for supply chain optimization. A single-size-fits-all AI model rarely works.
How to Avoid It
- Define critical business issues AI is supposed to address (e.g., minimizing churn, optimizing inventory, fraud detection).
- Establish quantifiable KPIs before AI deployment (e.g., improve forecast accuracy by 20%, cut manual reporting by 50%).
- Align AI projects with departmental and organizational goals.
- Implement pilot projects before expanding AI throughout the organization.
- Periodically assess AI performance against established benchmarks to guarantee alignment with objectives.
Pitfall #2: Inadequate Data Quality and Management

AI for business intelligence is only as good as the data it's fed. Faulty, incoherent, or skewed data results in spurious models and incorrect conclusions. Companies that use AI on raw, unstructured, or old datasets usually get their predictive models full of defects.
Why This Is a Problem
Inaccurate, missing, or incomplete data silos, inconsistencies compromise AI performance. The predictions will always be faulty if an AI model is trained on poor-quality data. Take the case of a bank integrating AI for credit risk rating. If the historical transaction information is incomplete or inaccurately labeled, the AI may misclassify borrower risk and make expensive mistakes.
How to Avoid It
- Implement strict data governance policies for consistency, accuracy, and completeness.
- Periodically audit datasets to remove inconsistencies and biases.
- Combine AI with data lakes and warehouses that centralize and cleanse data before analysis.
- Deploy data validation tools that mark errors before AI models process the data.
- Apply synthetic data augmentation methods to complete gaps in datasets.
- Train AI models on diverse datasets to reduce biases and improve generalizability.
Pitfall #3: Forgetting Human Expertise

AI may be able to process numbers at record speeds, but it does not possess domain knowledge, intuition, or contextual awareness. Companies using AI-based BI insights without human intervention will likely make uninformed decisions.
Why This Is a Problem
AI-driven decisions are made in a vacuum. For instance, a model might identify a sudden decline in revenue as unusual. Yet, only a seasoned finance team knows if it's because of seasonal patterns, regulatory updates, or market movement. Leave AI-generated reports without human intervention to mislead stakeholders.
How to Avoid It
- Use AI as a decision-support tool, not a decision-maker.
- Have human analysts approve AI-generated insights before making decisions on them.
- Foster cross-functional collaboration among AI experts and domain specialists.
- Create AI models with explainability capabilities to give a rationale for predictions.
- Train staff to accurately interpret AI insights and utilize them properly.
Pitfall #4: Disregarding Scalability and Integration Issues
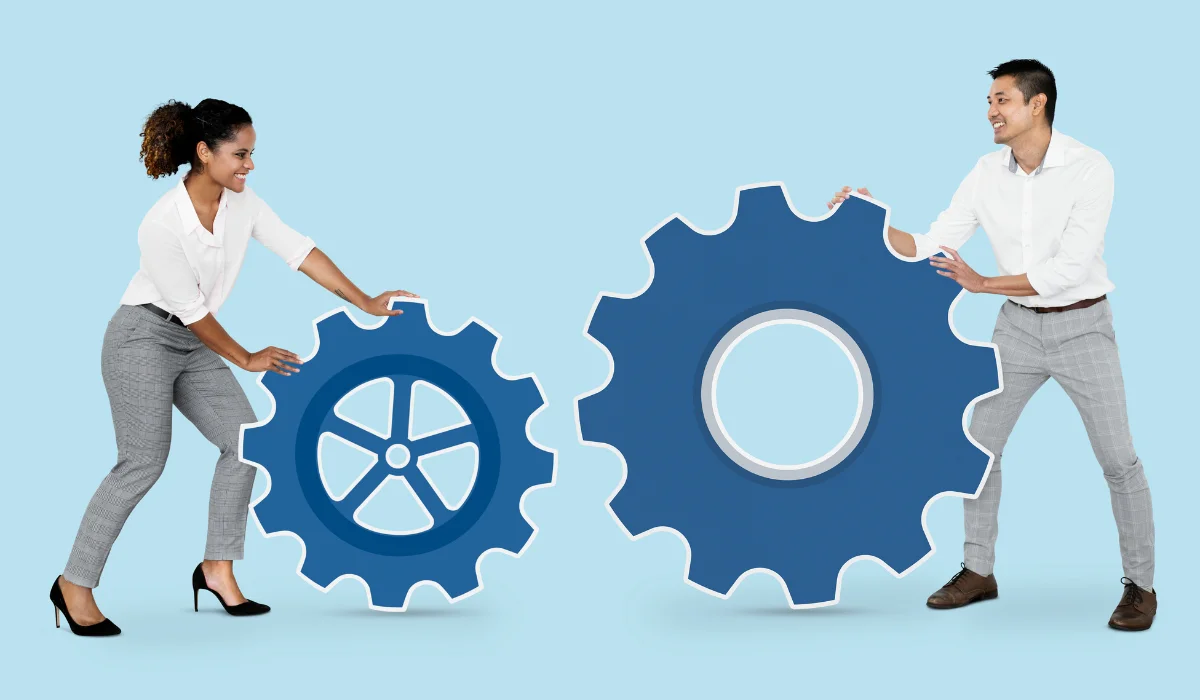
AI must integrate into current BI infrastructures without disrupting or bottling up. Most organizations do not pay attention to scalability and integration, resulting in inefficiencies and disintegrated workflows.
Why This Is a Problem
An AI solution that performs well in a pilot environment could collapse under enterprise-sized data loads. An AI product that won't plug into existing BI dashboards means teams must constantly switch back and forth between platforms, lowering productivity.
How to Avoid It
- Select AI solutions compatible with current BI products like Tableau, Power BI, or Looker.
- Prototype AI models in live conditions before scaling across departments.
- Design AI workflows to be flexible and scale up as data complexity increases.
- Invest in cloud-based AI platforms with elasticity in processing capacity and storage.
- Employ API-based architectures to allow seamless integration with existing BI systems.
Pitfall #5: Forgetting Ethical and Compliance Concerns
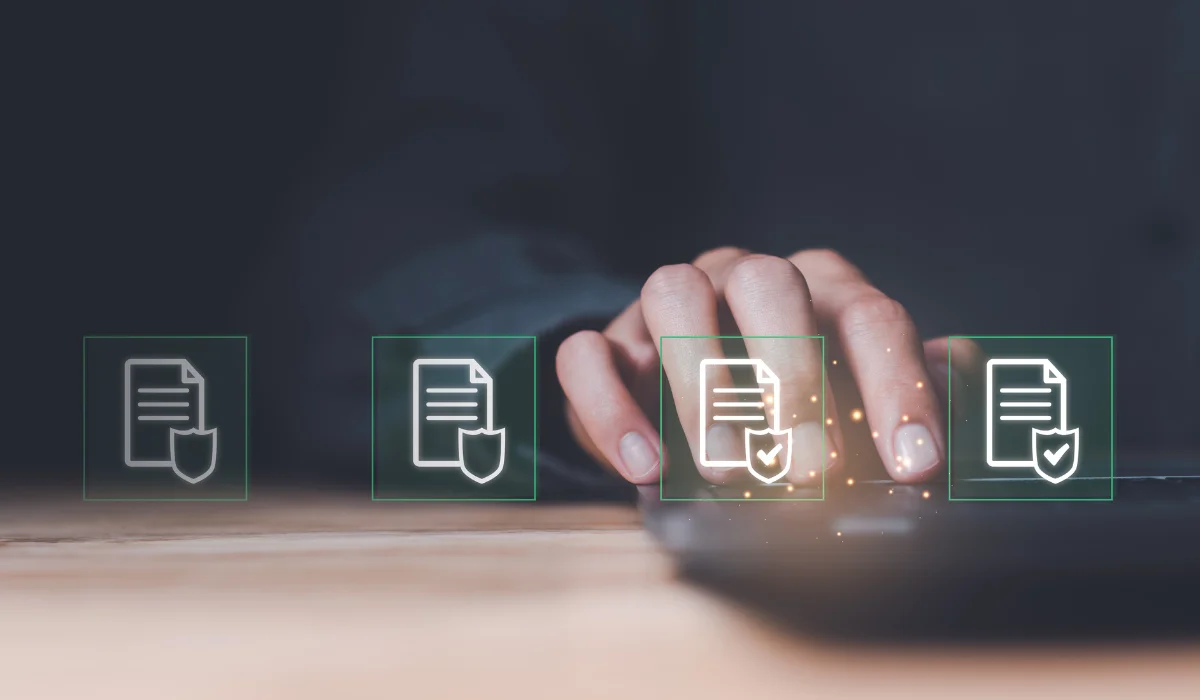
To integrate AI in business intelligence means handling sensitive customer data, financial transactions, and operational metrics. Mismanaging AI ethics or compliance can lead to legal consequences, reputational harm, and loss of consumer trust.
Why This Is a Problem
Regulatory agencies like GDPR, CCPA, and HIPAA have strict rules for AI-driven data processing. Failure to comply can attract huge fines and legal penalties. Black-box AI models that are not transparent also destroy trust in business decisions.
How to Avoid It
- Make AI-driven business intelligence solutions adhere to applicable data protection regulations.
- Employ explainable AI (XAI) methods to deliver transparency in AI-driven insights.
- Apply ethical AI frameworks to avoid bias and discrimination in analytics.
- Regularly audit AI to determine whether it is meeting legal and ethical requirements.
- Form AI ethics committees within organizations to manage responsible AI deployment.
Best Practices for a Successful Integration of AI in Business Intelligence
Aside from preventing pitfalls, companies should actively apply best practices to leverage AI in BI.
- Begin with clear AI use cases: Avoid fuzzy AI adoption; prioritize concrete applications with quantifiable ROI.
- Emphasize data integrity: Invest in data governance solutions that clean and normalize data before inputting it into AI models.
- Balance AI with human judgment: Use AI for automation but maintain decision-making within human hands.
- Ensure smooth integration: Select AI solutions to augment current BI tools and processes.
- Be compliant and ethical: Stay in sync with changing regulations and remain
- transparent in AI-powered analytics.
- Continuously monitor AI performance: Periodic checks allow fine-tuning models to achieve the best outcomes.
- Invest in AI education for staff: Empower teams to interpret and act on AI outputs effectively.
Conclusion
Companies that charge headlong into AI implementation without strategic vision tend to get bogged down in data chaos, operational inefficiency, and compliance issues. Steering clear of these five pitfalls-vague objectives, subpar data quality, excessive dependence on AI, scalability challenges, and ethical failings, ensures AI improves BI, not muddles it. With a systematic, well-thought-out strategy, firms can unleash the full power of AI, driving smarter, faster, and more trustworthy business intelligence insights. We hoped you have understood how to integrate AI into your business without failing.
Liked what you read?
Subscribe to our newsletter