How to Measure the Success of AI Business Intelligence: Key Metrics to Track
- SoftudeFebruary 24, 2025
- Last Modified onFebruary 24, 2025
Organizations increasingly integrate AI into their business intelligence (BI) platforms in today's data-driven era to glean actionable insights and achieve competitive advantage. As companies increasingly invest in technologies, evaluating their performance quantitatively is extremely important. This blog ventures into the parameters and methodology of evaluating AI performance in BI and takes a systematic step-by-step approach beyond casual observation.

AI in Business Intelligence
AI in BI is not merely a matter of automating what is already being done. It's about changing how data is interpreted and used. Most conventional BI systems rely on static reports and manual analysis of data. AI-driven BI, on the other hand, uses ML, NLP, and predictive analytics to provide dynamic insights that change as the data evolves.
For instance, where a traditional BI tool can report quarterly sales numbers, an AI-powered system can predict future sales based on the latest available data, seasonal variations, and market-related information. It is from this move from reacting to proactive decisions that the real value of AI in BI is anchored.
Why Success Measurement Matters
Success measurement of AI projects in BI matters for a variety of reasons:
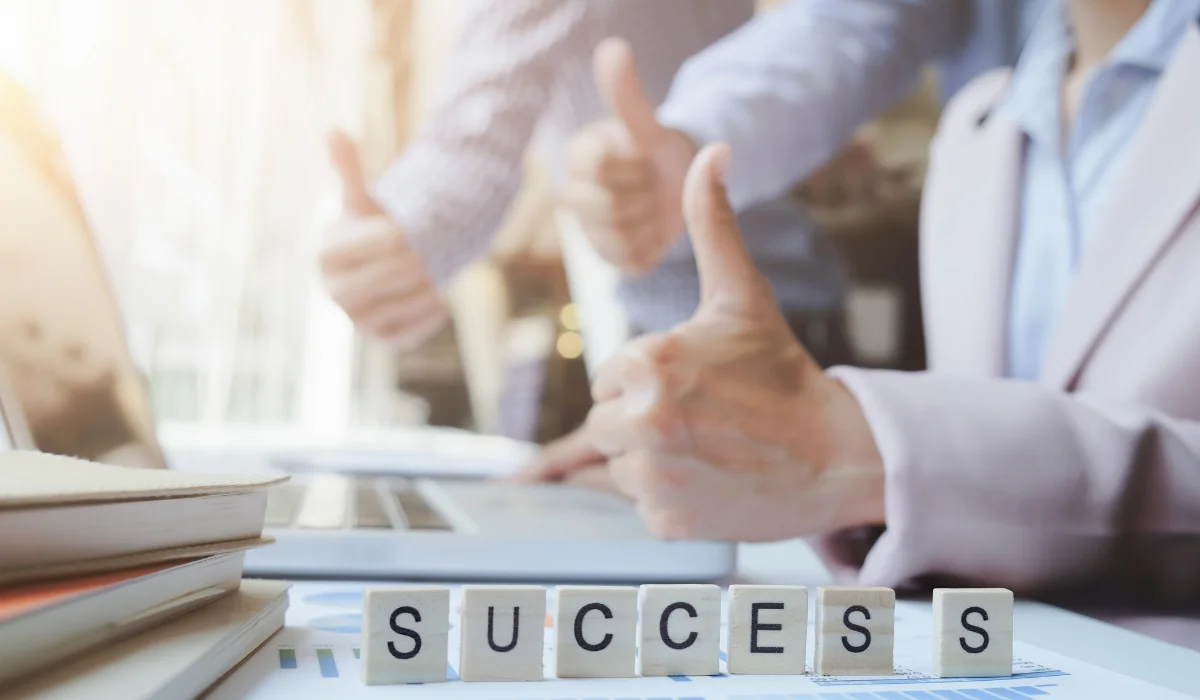
- Financial Accountability: Executives and investors need to be able to identify clear investment returns. Quantifying benefits such as cost savings and top-line improvements enables the ROI justification of AI initiatives.
- Operational Efficiency: By monitoring metrics such as process effectiveness and error reduction, organizations can look for areas where AI is improving operations and further tightening is needed.
- User Adoption: High user adoption rates indicate the technology is extremely usable and well incorporated into everyday work processes. This is essential to long-term success.
- Strategic Planning: Quantifiable metrics allow leadership to incorporate AI projects into business strategy, ensuring technology investments inform long-term plans.
Without robust measurement systems, companies can risk overstating the value of AI or losing the opportunity to improve key processes.
Key Metrics to Track: The Ultimate KPI Playbook
A structural approach to measurement necessitates that the metrics be divided into various categories. Each category emphasizes different aspects of the performance and impact of the technology.

1. Return on Investment (ROI)
ROI is simple. The cost of implementing AI is divided by the revenue or cost savings it generates. If an AI project costs $200,000 and generates $600,000 in new revenue or cost savings, the ROI is 200%. This metric directly confirms both the initial and ongoing investments in AI.
2. Revenue Growth
It's important to track changes in revenue that can be directly linked to AI programs. Whether creating new revenue streams, upsell opportunities or customer retention; careful analysis can separate the impact of AI from other market influences.
3. Cost Savings
Measure cost savings in operation. For example, by automatically processing routine data tasks, an organization can reduce labor costs or eliminate costly errors. Documenting the savings demonstrates tangible financial returns to be achieved in deploying AI technology in BI systems.
4. Efficiency Gains
Measure how AI boosts workflow productivity. A good benchmark is the reduction in time required for manual tasks. If one reporting process, which used to take 10 hours a week, now only takes 2 hours, then that's a tremendous productivity boost.
5. Error Rate Reduction
Monitor the decrease in data errors following the integration of AI. By looking at historical error rates compared to current rates, you can determine if the new system offers better data accuracy, which is vital for making proper decisions.
6. Cycle Time Reduction
Track how quickly AI processes and delivers data insights compared to the traditional method. Quicker cycle times can lead to faster decision-making, giving businesses a competitive edge.
7. Predictive Accuracy
Predictive models are only as useful as their validity. Track statistics like precision, recall, and F1 scores to assess how well AI predictions match true outcomes. Steady increases in accuracy show a good, well-tuned model.
8. Model Performance
Daily checks using loss functions, accuracy rates, and confusion matrices give an in-depth look at the effectiveness of the AI algorithms. Insights are crucial for incremental refinement over time.
9. System Uptime
Track the reliability and availability of your AI systems. Consistent downtime will disrupt business processes and erode trust in the technology. High uptime percentages must be maintained to allow for uninterrupted business processes.
10. Adoption Rates
Track the pace at which and the degree to which the new AI tools are being utilized across the organization. Low adoption may indicate usability issues or a lack of training,
whereas high adoption indicates that the technology is seamlessly integrated.
11. User Satisfaction
Collect feedback through surveys, interviews, or focus groups. Understanding the effect of AI tools on daily work and where they can be enhanced will give valuable qualitative feedback to complement quantitative data.
12. Training Effectiveness
Measure how effectively your employees understand the AI tools through tests or changes in performance following training. Effective training translates into effective use of technology and maximizes its overall impact.
14. Data Integrity
Ensuring the information driving your AI solutions is reliable, complete, and accurate is paramount. Flawed or changing data can skew insights and degrade the overall performance of AI algorithms.
15. Timeliness
Measure the recency of your data. Real-time or near-real-time data is critical to accurate predictions, so monitoring data refresh cycles is key.
16. Consistency
Verify that data standards are maintained consistently in different sources. Consistent data gathering and processing are the foundation for establishing sound comparisons and wise decisions.
Tools & Techniques for Successful Measurement

Deploying AI is only half the battle, the other half measures its effect successfully. Several tools and techniques can be used to streamline this process:
Dashboards and Analytics Platforms
New-generation BI solutions provide interactive dashboards that bring together KPIs in real-time. These platforms enable stakeholders to view performance at a glance, instantly recognizing trends and outliers.
Benchmarking
Before implementing AI solutions, baseline measurements need to be put in place.
Benchmarking offers a baseline against which pre- and post-implementation performance can be measured, marking the areas improved and possibly where the AI model is not performing as desired.
Qualitative Feedback
Numbers give half the picture, but qualitative feedback fills in the gaps. Surveys and interviews with end users regularly can reveal usability flaws, training inadequacies, and unintended advantages that raw numbers may fail to demonstrate.
Data Visualization
Good visualization converts intricate datasets into consumable insights. Charting, graphing, and heat maps are useful tools for identifying trends, comparing performance by time, and easily detecting abnormalities that may be missed otherwise.
Conquering Common Measurement Challenges
Despite having the appropriate metrics, several challenges can make the measurement process more difficult:

Data Bias
AI models rely on the quality of input data. Biases in unbalanced sample sizes, stale data, or unreliable data sources can result in poor predictions. Periodic audits and verification of data sources are required to ensure data integrity.
Interpreting Raw Numbers
Metrics are only as good as the context in which they are used. A high predictive accuracy, for example, may sound impressive until you learn that the data it is based on is skewed. Using quantitative metrics in conjunction with qualitative observations provides a more nuanced perspective.
Stakeholder Alignment
Various departments may have different priorities. These various needs under one measurement framework call for effective communication and standard reporting procedures. Involving stakeholders early on can assist in defining what success is for the organization.
Conclusion
Gauging the effectiveness of AI for business intelligence is as much an art as a science. It involves having a good idea of what your company wants to accomplish, being steadfast in the importance of data quality, and adjusting as the technology changes. By prioritizing financial, operational, performance, user-centric, and data quality metrics, you can create a solid framework that will justify your spending and continually improve.
If your company is looking into AI projects or trying to improve current systems, audit your current BI processes. Involve your staff to set baseline measures, collect quantitative data and qualitative information, and create recurring review cycles to measure performance. These will ensure that your AI investments pay off in terms of concrete business value.
Liked what you read?
Subscribe to our newsletter