10 Must-Do Practices for Practical AI Implementation
- SoftudeFebruary 21, 2025
- Last Modified onFebruary 24, 2025
Merging AI into business intelligence is no simple upgrade. It requires a disciplined, systematic approach. This guide outlines ten best practices for AI implementation to guarantee that your organization deploys AI with precision, accountability, and technical expertise.
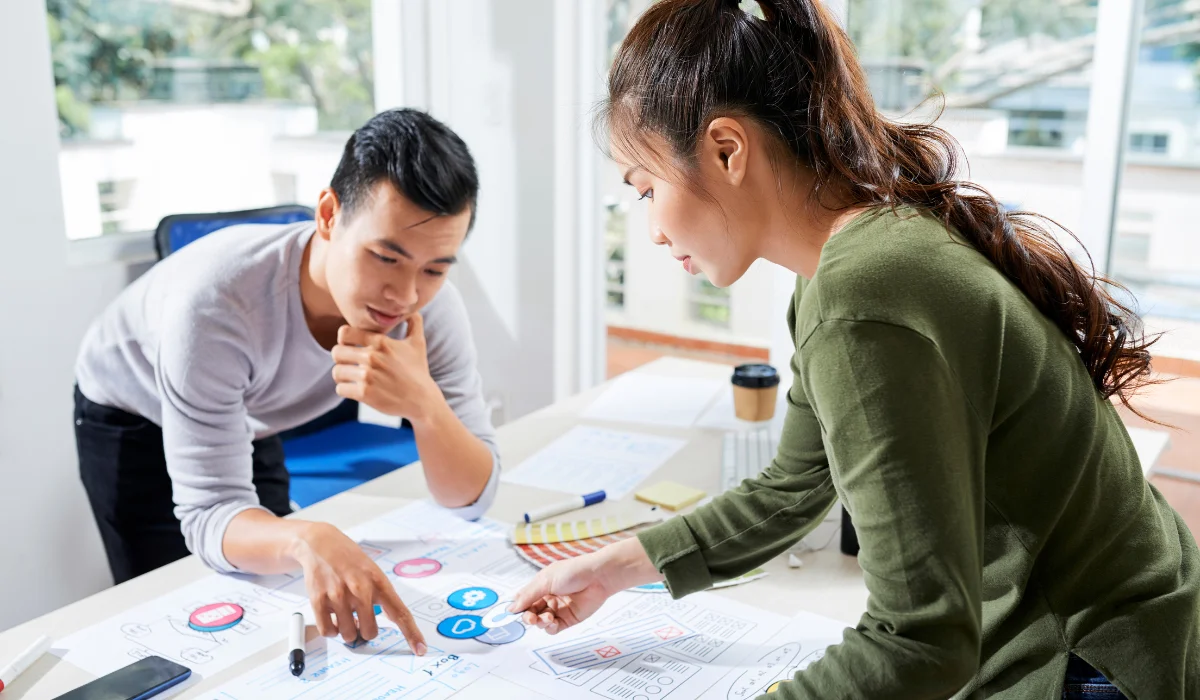
Practices and Strategy for Successful AI Implementation in Your Business
1. Set Clear Oversight Structures
A strong AI approach starts with a good governance framework. Creating control structures involves establishing special committees or executive positions devoted entirely to AI projects.

Such bodies should be able to scrutinize project proposals, fund allocation, and risk management. A good governance framework imposes stringent standards, provides the foundation for AI initiatives, and ensures that technical innovation is aligned with strategic objectives.
Key insights:
- Risk Management: Establish risk analysis procedures for biases or unforeseen consequences.
- Strategic Alignment: Ensure regulatory bodies are linked to high-level management, so AI projects align with the company’s strategy.
2. Define Roles and Responsibilities for AI Governance

Clear roles prevent overlap and ensure accountability. Outline each job, from managing data to overseeing technical work and meeting regulations. Document responsibilities for data scientists, engineers, compliance officers, and business analysts in an organized way.
A formal RACI (Responsible, Accountable, Consulted, and Informed) matrix may be a handy utility in this case, making it clear to all stakeholders what role they have in the AI lifecycle.
Key takeaways:
- Specialization: Delegate work based on competency to avoid miscommunication and effort duplication.
- Cross-Departmental Collaboration: Develop an environment where technical groups and business units collaborate, closing the gap between data and decision-making.
3. Develop Decision-Making Procedures for AI Strategy
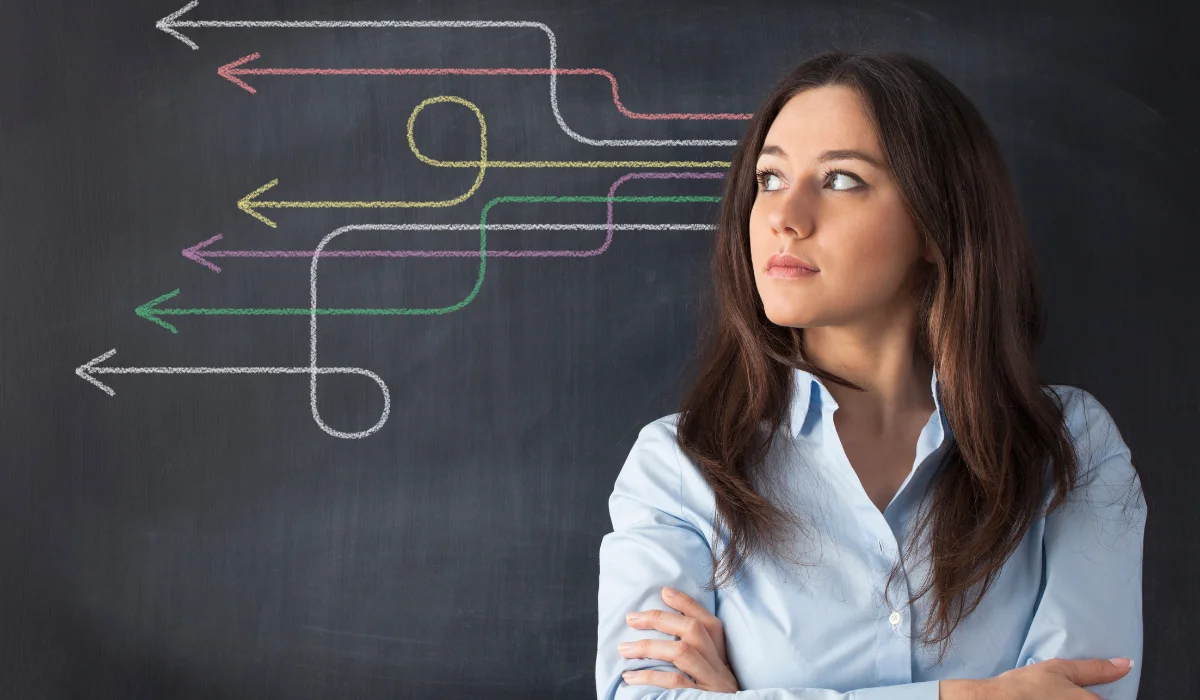
Informed decision-making is essential for practical AI implementation. Implement formal processes that govern the evaluation, approval, and execution of AI projects. This involves setting project selection criteria e.g., ROI, scalability, and integration with current systems and implementing recurring review cycles.
Decision-making processes should focus on data-driven metrics and openness. A properly documented process minimizes the chance of subjective bias and ensures each project aligns strategically with the organization's goals.
Key insights:
- Evaluation Metrics: Employ measurable indicators to determine potential benefits and risks.
- Documentation: Keep extensive records of decisions to assist in audits and post-implementation reviews.
4. Hire Technical Experts with AI Experience

The success of AI deployment depends on the quality of your technical team. Hire professionals with experience in machine learning, data science, and software engineering.
The team must be skilled in managing large data sets, implementing machine learning models, and ensuring algorithms run smoothly under different conditions. Instead, think of a combination of hiring and internal upskilling to create a team that not just gets the technical stuff but can incorporate AI into more comprehensive business processes.
Key insights:
- Continuous Learning: Create programs for continuous technical advancement to stay in sync with fast rates of AI development.
- Technical Rigor: Emphasize candidates with a history of creating scalable, reliable AI systems in real-world settings.
5. Involve Business Leaders in AI Strategy
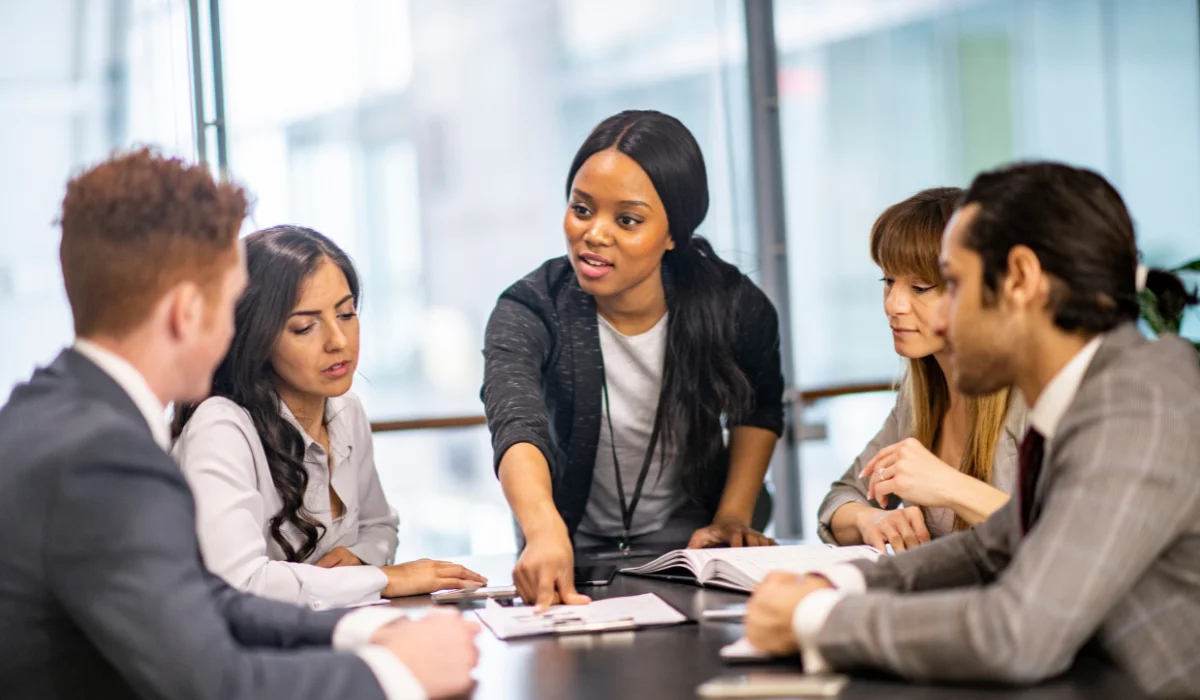
Business goals should drive technical innovation. Engage business leaders early in AI planning to ensure projects address genuine market requirements and deliver quantifiable value.
These leaders can offer insights into customer behavior, market direction, and financial goals to ensure AI projects drive tangible results. Their strategic guidance is essential to prioritize projects and allocate needed resources.
Key insights:
- Strategic Integration: Business executives should periodically scrutinize AI project results against overall corporate objectives.
- Resource Allocation: Their intervention aids in reconciling the technical aspirations with viable budget and operational limitations.
6. Hire Ethics Reviewers for Accountable AI
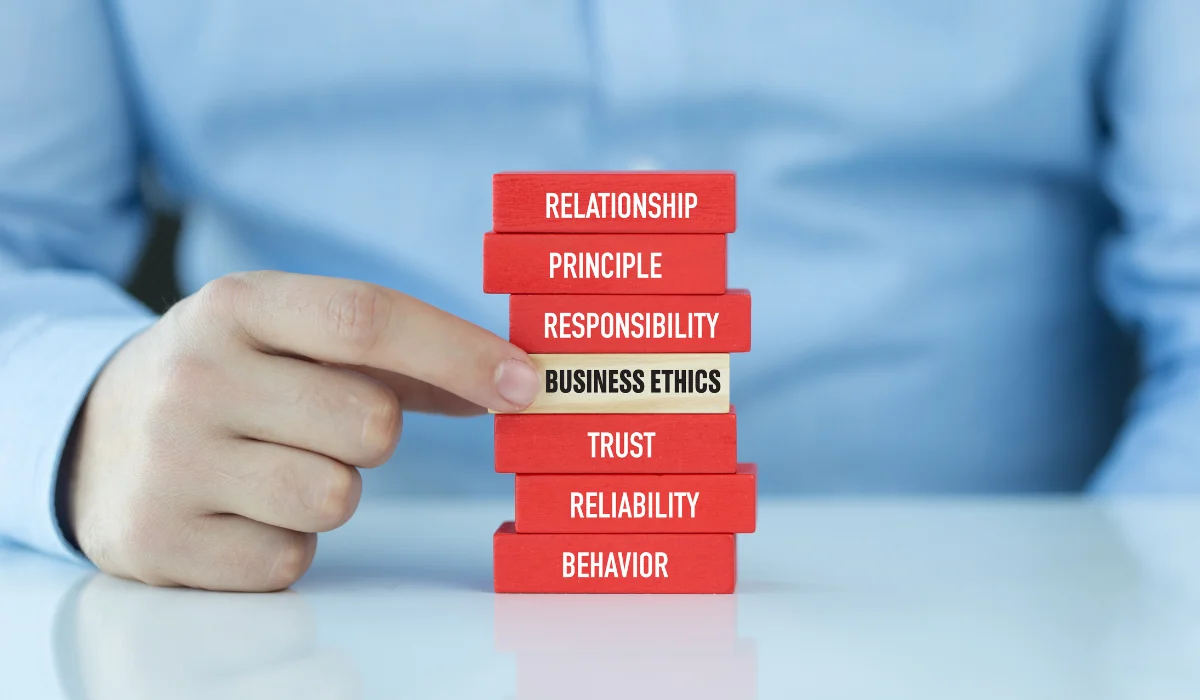
AI systems inherently have ethical implications. Bring ethics reviewers into your governance structure to regularly review AI applications for fairness, accountability, and transparency. They assess whether the AI systems meet legal requirements and ethical standards, ensuring that decisions are transparent and unbiased. Incorporating ethical reviews early in the design stage prevents potential reputational and operational risks.
Key insights:
- Bias Mitigation: Conduct systematic reviews to identify and address biases in data and algorithms.
- Transparency: Create protocols enabling transparent explanation of AI-driven decisions to internal stakeholders and external regulators.
7. Engage End-User Representatives for Practical Insights

Get input from the people who use AI systems every day. End-user representatives can provide valuable feedback on usability, performance, and how well the AI fits into daily work. Their insights help ensure that AI solutions are not only technically sound but also practical and easy to use.
Principal insights:
- User-Centric Design: Eagerly collect end-user opinions to tailor system interfaces and functionality.
- Iterative Improvement: Utilize actual use data to inform ongoing improvement in AI usage.
8. Establish Performance Monitoring and Periodic Audits
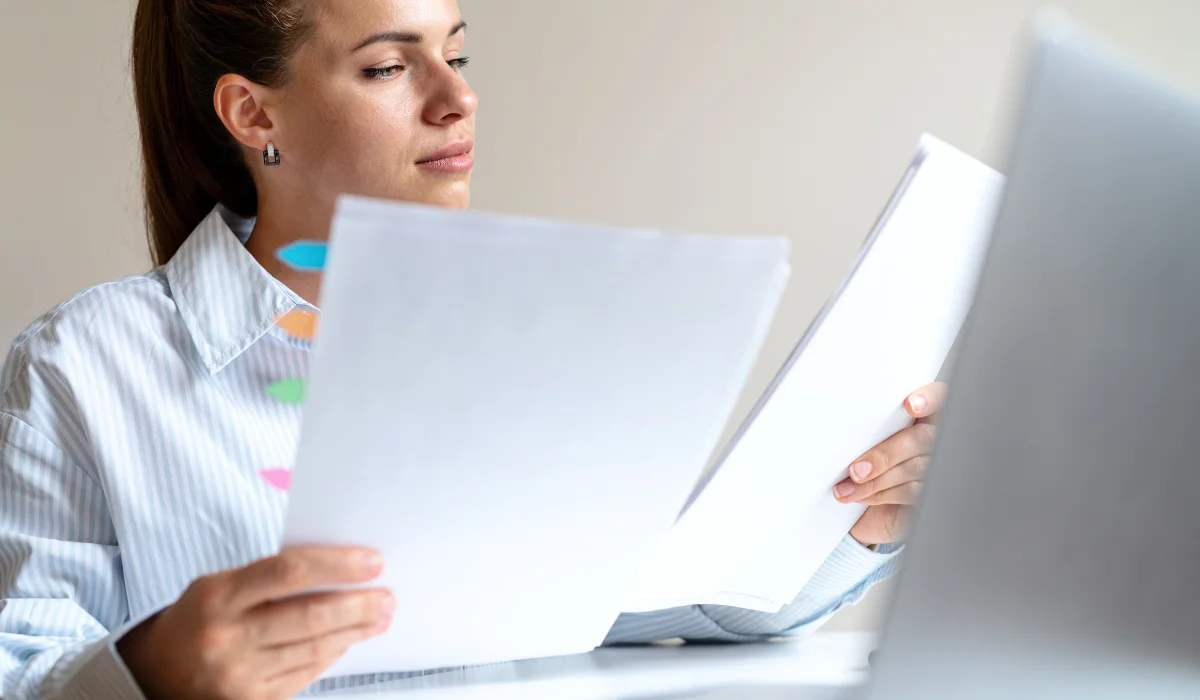
Successful AI systems need continuous assessment. Install performance monitoring software that tracks critical performance indicators (KPIs) like accuracy, latency, and user interaction.
Periodic audits (technical and procedural) are needed to ensure that the AI models are performing as designed and in line with business goals. The audits should also evaluate the strength of security measures and adherence to regulatory requirements.
Key insights:
- Data-Driven Adjustments: Leverage audit findings to refine models and rebalance strategies.
- Transparency and Accountability: Document audit procedures and make them accessible for internal scrutiny or external examination.
9. Ensure Strict Compliance Checks and Gather User Feedback
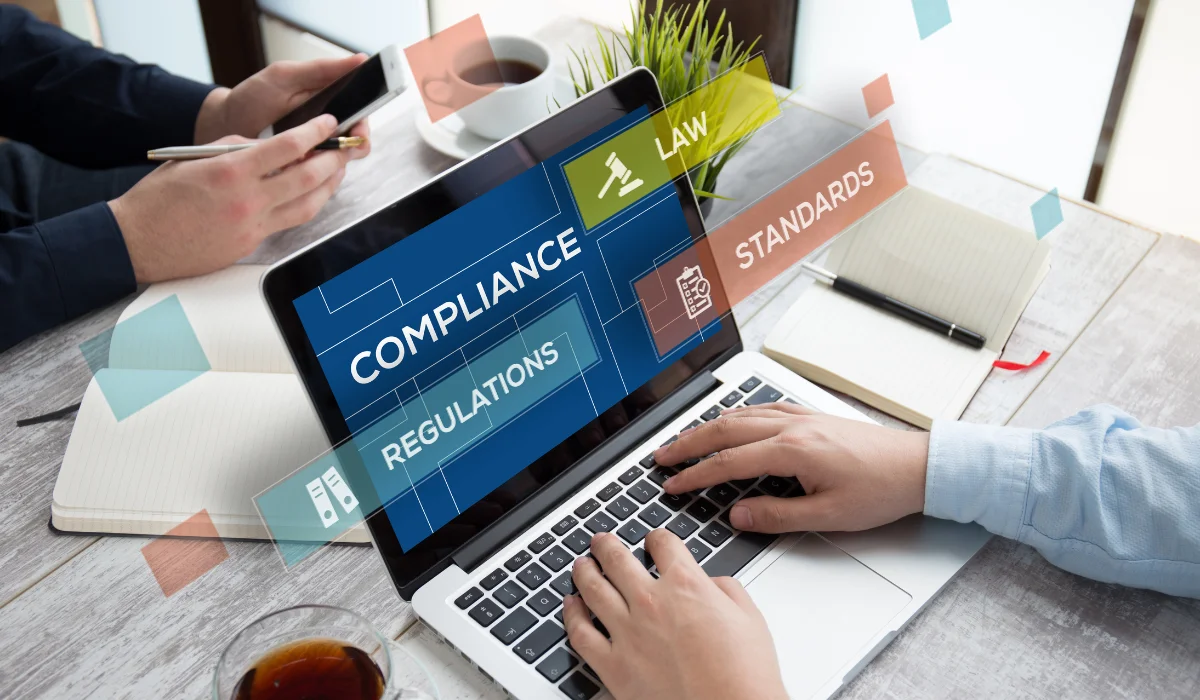
Compliance is not fixed; it must adapt to regulatory and technological developments. Implement strict procedures for compliance audits that guarantee compliance with data privacy, security requirements, and sectoral regulations.
Concurrently, establish processes to gather and analyze user feedback systematically. This two-pronged approach ensures regulatory compliance and creates a culture of ongoing improvement, wherein the user experience informs incremental enhancements.
Key insights:
- Regulatory Awareness: Keep up with changing regulatory needs and modulate compliance infrastructure accordingly.
- Integration of Feedback: Create formal feedback channels to collect actionable information from end users for further system refinement.
10. Construct an Integrated Training Program for All Levels

A thorough training program is the key to long-term AI integration in your business. Create tiered training beginning with basic AI literacy for every employee, progressing to advanced technical training for specific positions, and adding leadership training for strategic guidance.
Periodic refresher courses will keep the workforce proficient in managing new tools and methods. This forward-looking investment in human capital is as important as the technology itself.
Key insights:
- Scalability: Make training programs scalable and flexible to adapt to technological changes.
- Practical Application: Integrate practical exercises and actual case studies to link theory and practice smoothly.
Conclusion
In today's competitive environment, successful business intelligence integration of AI relies on more than technological prowess. It requires disciplined governance, defined roles, and continuous learning. Through the creation of strong oversight, defining responsibilities, codifying decision-making processes, and investing in technical and ethical expertise, organizations can develop AI systems that are effective and resilient.
Implement these ten best practices for AI implementation for measurable and strategic success. With thorough performance monitoring, compliance audits, and sustained training, your organization will be fully capable of leveraging the transformative power of AI responsibly and sustainably.
Liked what you read?
Subscribe to our newsletter