The Fine Line Between AI Agents and AI Models
- SoftudeApril 11, 2025
- Last Modified onApril 11, 2025
Do you also assume AI agents and AI models are the same? Many AI vendors and industry experts use them interchangeably, so it is obvious to think that way. However, there is a fine line between the two. Treating them as one and the same might result in inefficiencies, missed opportunities, or, worse, like you might implement the wrong solution to the problem at hand. Therefore, it is better to understand the difference between AI agents and AI models.
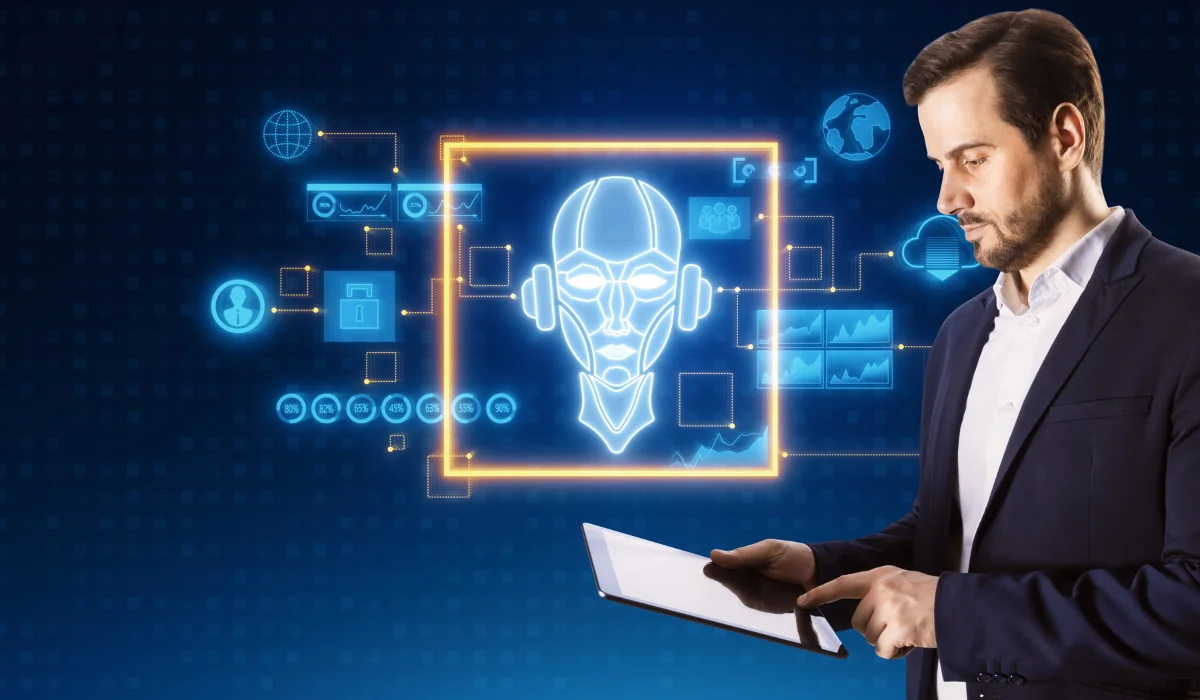
So, let's take a closer look at how these two differ at their core and why that distinction could greatly impact how your business navigates the AI landscape.
What is an AI Model?
Simply put, an AI model solves highly specific, well-defined problems by analyzing data. Whether using a deep learning model to predict stock prices or a machine learning model to categorize customer feedback, you rely on a data-driven construct to make predictions or classifications based on a fixed set of inputs.
Yet, an AI model is in a finite domain. It does not naturally adapt to novel challenges or contexts, it must be constantly retrained to remain pertinent. Deployed, it's fixed, constrained by the data set it was trained on. AI models perform best in forecasting applications where prior patterns are crucial to future behavior.
The Advantages of AI Models
- Accuracy in Forecasting Tasks: When it comes to forecasting, predicting trends, or analyzing past data, AI models can perform better. Their capacity to handle huge chunks of structured data enables companies to make extremely accurate predictions regarding future occurrences.
- Task Automation: AI models are well-suited to automate repetitive, data-intensive tasks. This may range from detecting fraud in financial transactions, sorting emails according to their content, or identifying potential risk factors in healthcare data.
- Scalability: Once trained, AI models can scale easily and handle larger datasets, making them well-suited for industries that must deal with increasing data volumes, such as e-commerce, social media, or health tech.
AI Models Are Defined by Their Limitations
While their accuracy can be phenomenal, AI models are generally restricted by the training setup. They don't learn or transform unless specifically retrained on fresh data. One thing to recognize here is that AI models lack the ability to act independently, they just take inputs and produce predictions or outputs.
For example, an AI model deployed in the retail industry may forecast customer buying patterns based on experience. But what if the market environment suddenly alters? The model will not be able to recognize such changes unless it's directly retrained with new data that incorporates those changes.
What are AI Agents?

Where AI models process data, AI agents go a step further. These are dynamic, autonomous systems designed to interact with their environment, make decisions, and take action based on real-time data. Unlike AI models, which are passive, AI agents are goal-driven and capable of navigating complex, unpredictable environments.
Think of an AI agent as a decision-maker: it senses the environment, processes the information, and makes decisions in pursuit of specific objectives. AI agents are typically built using techniques like reinforcement learning (RL), where they learn from both successes and failures in their environment and continuously optimize their decision-making strategies.
The Gains With AI Agents
- Adaptability: Perhaps AI agents' greatest strength is their real-time adaptability. In domains where conditions shift continuously, such as autonomous vehicles or smart customer service, AI agents can rapidly respond and adapt their behavior to maximize performance.
- Actionable Insights and Autonomy: AI agents may act directly on the insights they derive from inputs, which makes them ideal for scenarios that call for autonomous decision-making,e.g., dynamically rerouting shipments in logistics or answering customer queries in customer service systems.
- Continuous Learning: AI agents learn and evolve. Through feedback loops, they update their actions based on the success or failure of their decisions, continuously improving over time without the need for human interference.
Key Industry-Specific Applications of AI Models
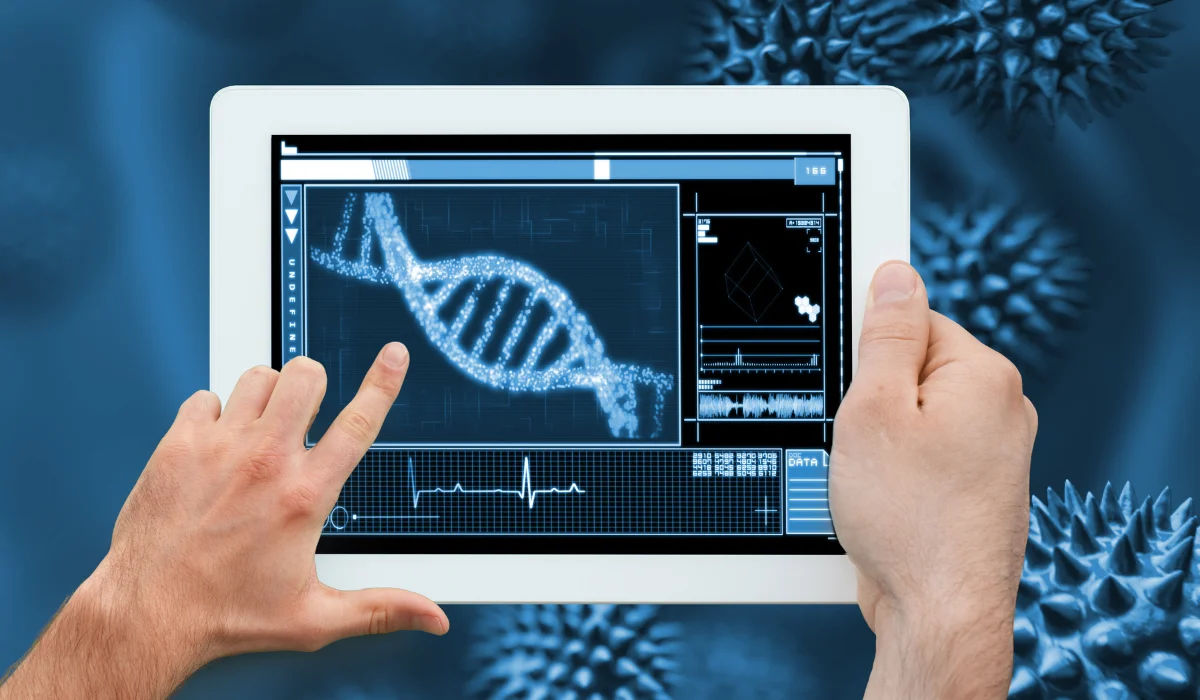
AI models are most important in sectors where historical data analysis is a key function in business. The models work best in conditions that need precision and crisp predictive analytics, especially when the problem being solved is well defined.
1. Healthcare: Medical Diagnosis and Disease Prediction
In the field of healthcare, AI models find widespread application for forecasting patient outcomes or disease diagnoses from past medical histories, gene information, and imaging. As an example, machine learning algorithms trained on enormous sets of patient histories can estimate the probability of a patient acquiring diabetes or cardiac disease. They examine previous patients' data in order to provide early detection tools and assist in treatment planning with healthcare providers.
2. Finance: Credit Scoring and Fraud Detection
In the financial industry, AI models cannot be avoided in activities such as credit scoring and fraud detection. For example, machine learning models are employed by banks to evaluate the credit rating of prospective borrowers. The models review past spending habits, history of transactions, and market behavior to forecast future behavior. Equally, AI models assist in detecting fraud through the identification of abnormal patterns that diverge from set norms.
3. E-Commerce: Personalized Product Recommendations
E-commerce giants like Amazon and Netflix use AI models of recommendation engines. Through the analysis of historical user behavior, like past buying patterns or content viewing, AI models forecast a user buying a given product or interacting with specific content. These forecasting models drive individualized user experiences, which elevate customer satisfaction and sales conversions.
Key Industry-Specific Use Cases of AI Agents

AI agents excel in domains where independent decision-making and timely adaptability are essential. They are ideal for settings that demand the agent to interact with and react to ever-changing information.
1. Autonomous Vehicles: Self-Driving Cars
Autonomous vehicles employ AI agents to drive and make decisions using real-time sensor data. These cars need to interact with their surroundings, decide on obstacles, traffic, and speed, and adapt to changing road conditions. An AI agent here employs reinforcement learning to maximize driving strategies, improving performance in every driving encounter.
2. Supply Chain and Logistics: Dynamic Inventory Management
AI agents are an integral part of inventory management in dynamic settings such as warehouses and logistics systems. For example, intelligent warehouses utilize AI agents to dynamically optimize inventory levels in real-time, adapting inventory to changes in demand, arriving shipments, or sales volumes. These agents are not fixed but continually adapt their behavior to real-time information, such as redirecting shipments when some items are out of stock or restocking shelves based on anticipated future demand.
3. Customer Support: Smart Chatbots and Virtual Assistants
Most businesses now use AI agents as smart chatbots or virtual assistants. These agents can take sophisticated customer support requests on their own, processing customer questions and responding accordingly, booking an appointment, taking an order, or resolving a problem. As these AI agents continue to interact, they learn from the process and adapt their responses based on feedback in real-time.
AI Agents Vs AI Models: Understanding the Technological Divide
Understanding these differences between AI agents and AI models are essential when selecting the right AI solution for your business:
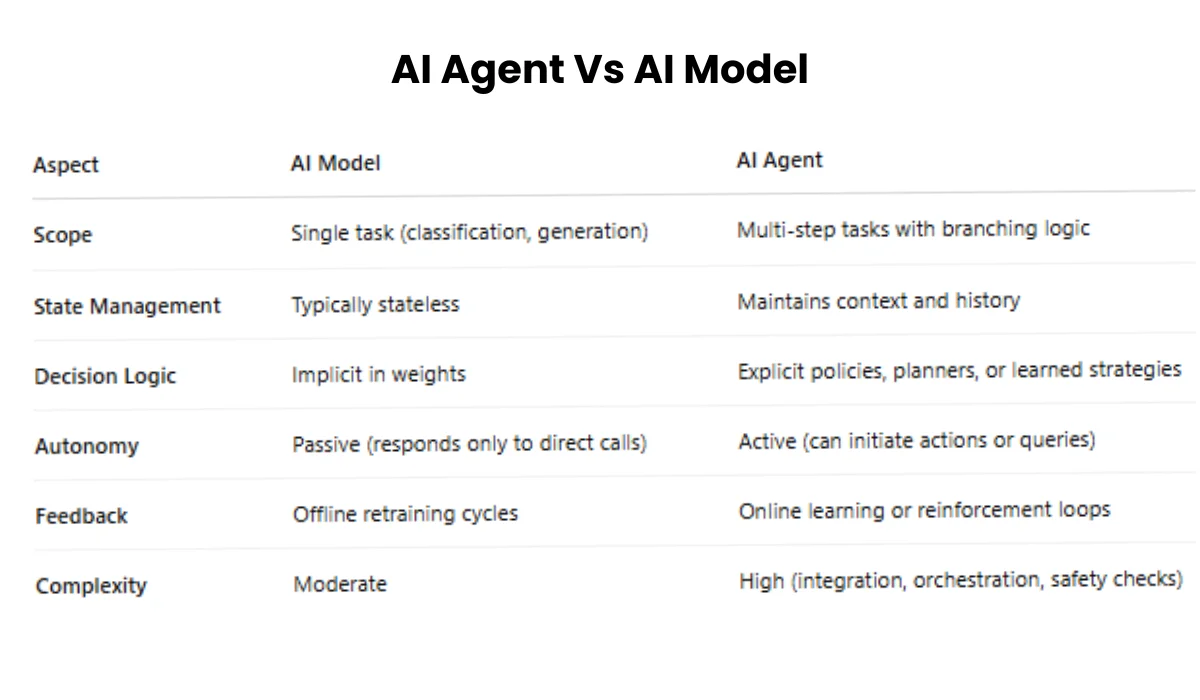
Differences Matter, Here’s Why?
The key takeaway here is that AI models and AI agents serve fundamentally different roles. AI models will likely suit your needs if your business is looking to implement predictive analytics, optimize customer segmentation, or streamline specific tasks like fraud detection or demand forecasting. They are precision instruments trained to deliver outcomes within a defined scope.
On the other hand, if your business operates in an environment where decisions need to be made on the fly, where the landscape is unpredictable, and adaptive decision-making is essential, AI agents are the solution. These agents excel in contexts such as autonomous vehicles, intelligent customer service systems, and real-time resource allocation in industries like manufacturing or logistics.
Final Thoughts
Choosing between an AI agent and an AI model is not just a technical decision; it’s a strategic one. Your choice will depend on the level of autonomy required, the nature of the problems you are trying to solve, and the environment in which the AI will operate. By understanding these key distinctions, you’ll be equipped to make a more informed decision on how to leverage AI in your business and ensure that the technology you implement can deliver tangible, long-term value.
Liked what you read?
Subscribe to our newsletter