How Machine Learning is Powering Smarter AI Agents
- SoftudeApril 11, 2025
- Last Modified onApril 11, 2025
In the fast-changing times of artificial intelligence, AI agents are becoming key elements of ordinary technologies- from custom virtual assistants and chatbots to self-driving robots and smart recommendation systems. It is not simply a collection of pre-programmed instructions that enable these agents to act intelligently, but also the incorporation of machine learning (ML).

In this blog, we’ll explore the transformative role of machine learning in AI agent development, explain how it fuels intelligence in machines, and examine the specific ways machine learning is applied to empower AI agents.
What Are AI Agents?
An AI agent is a computer program that senses its environment, processes the information it perceives, makes decisions, and acts towards achieving certain goals. In contrast to routine computer programs based on static rules, AI agents learn and improve over time, owing to machine learning.
These agents are programmed to function autonomously or semi-autonomously in changing environments. Whether it is a customer support chatbot responding to customer inquiries or an autonomous vehicle driving through traffic, AI agents heavily depend on machine learning to execute efficiently.
How Machine Learning Augments AI Agents

Let's consider how AI agent creation with ML makes these agents see, learn, adjust, and act intelligently in real-life situations.
1. Perception and Environment Understanding
AI agents must learn to perceive their environment so they can respond suitably. Perception is constructed through the processing of input from different sources such as images, text, speech, or sensors.
- Natural Language Processing (NLP): Through machine learning, AI agents gain the ability to process human language. NLP enables agents to read meaning from written or spoken text so that they can respond to questions, translate languages, or engage in conversation like humans.
- Computer Vision: Agents can process visual information thanks to ML algorithms. This is essential in uses such as facial recognition, medical image processing, and object recognition in autonomous vehicles. Machine learning is needed to provide visual perception beyond rudimentary rule-based recognition.
- Speech Recognition: Machine learning algorithms are taught to recognize patterns from audio and transcribe spoken words into text. That is what enables voice-operated assistants to process commands in real time.
Such abilities enable AI agents to converse naturally with people and adjust to a wide range of inputs, simulating human-like sensory perception.
2. Learning and Adaptation
One of the fundamental strengths of machine learning within AI agents is the capacity for learning from the past and refining their behavior according to it.
- Supervised Learning: Here, AI agents learn from labeled sets where the accurate outputs are pre-known. An example is training an email spam filter using a thousand emails classified as "spam" or "not spam" to learn correctly how to mark future messages appropriately.
- Unsupervised Learning: This approach enables agents to examine unlabeled data and find underlying patterns or clusters. It's helpful in situations such as customer segmentation or anomaly detection, where the agent needs to identify trends or unusual activity without direct guidance.
- Reinforcement Learning: This is error-and-trial-based learning where agents engage with an environment, receive feedback in the form of rewards or penalties, and learn the best strategies in the long run. Reinforcement learning has extensive applications in robotics, game playing, and real-time decision systems such as self-driving cars.
By these learning methods, AI agents grow more intelligent every minute, improving accuracy, efficiency, and performance as time goes on.
3. Decision-Making and Planning

Another essential function of ML in AI agents is to enable informed and strategic decision-making. AI agents tend to need to analyze information, weigh various options, and select the optimal action to meet their objectives.
- Predictive Modeling: Machine learning enables AI agents to predict future outcomes based on past information. For instance, a virtual investment advisor can employ predictive models to project stock trends and suggest investment strategies.
- Optimization Algorithms: ML algorithms are utilized by AI agents to decide on the most cost-effective or profitable route in any scenario. In supply chain management, for instance, AI agents can shorten delivery routes, lower operational costs, and enhance logistics workflows.
- Simulation and Strategy: Agents in complicated environments can simulate various situations to predict the outcomes of their actions. This facilitates improved long-term planning and risk management, such as in AI use in medicine, where treatment plans are simulated before recommendation.
Machine learning provides AI agents with the mental capabilities required to manage uncertainty, complexity, and dynamic environments effectively.
4. Personalization and User-Centric Interactions
Personalization is now a standard user expectation, and machine learning for AI agents is what enables this level of customized experience.
- User Behavior Analysis: AI agents continuously collect and analyze data about user preferences, actions, and interests. This enables them to customize responses and suggestions effectively. Think of how Netflix suggests shows based on your viewing history- it’s a machine learning-powered agent at work.
- Dynamic Content Delivery: Rather than presenting the same message to all users, AI agents personalize marketing messages, alerts, and product recommendations based on individual behavior and demographics.
- Context Awareness: Powerful AI agents don't simply react to what a user says, they know why it was said, based on the context. This allows for more insightful communication and effective problem-solving.
This degree of personalization creates greater interaction and user satisfaction, making AI agents an asset in customer-facing applications.
5. Self-Operating and Ongoing Improvement
AI agents powered by machine learning can improve and operate independently without any intervention from humans.
- Self-Training: AI agents can detect when they are performing poorly and train themselves with new data. For example, fraud detection agents in banking systems refresh their models automatically when new patterns of fraud are discovered.
- Anomaly Detection: Agents learn to recognize normal behavior and detect unusual patterns without pre-specified rules. This is particularly helpful in cybersecurity, where agents can identify breaches or threats in real time.
- Scalability and Flexibility: With machine learning, AI agents can be scaled across various domains with little reprogramming. An agent that has been trained to read customer sentiment in retail can be repurposed to perform in healthcare or education, given the proper data.
With these ability forces, AI agents are consistent, scalable, and able to perform in rapidly changing situations.
Challenges in AI Agent Development with ML
Even with its great potential, AI agent development with ML presents some challenges.
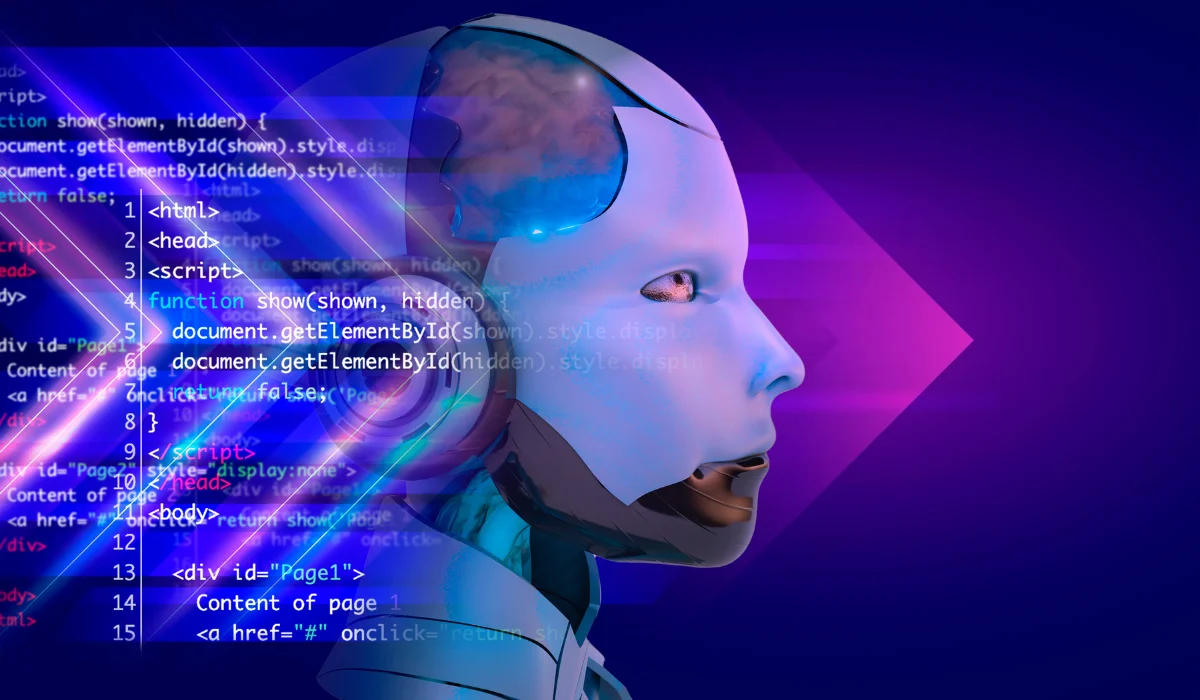
1. Data Quality and Quantity
Training machine learning models need to process massive amounts of high-quality data. Low-quality or incorrect data will result in wrong decisions and low agent performance.
2. Computational Demands
Machine learning and deep learning training, and processing them, might be computationally intensive, making special hardware and cloud resources a requirement.
3. Transparency and Explainability
It's usually unclear why a machine learning model came to a particular conclusion. In sensitive domains such as law or healthcare, this lack of visibility can be a significant roadblock to adoption.
4. Ethical and Security Concerns
With more user data being processed by AI agents and with them making independent decisions, it's essential to ensure fairness, data privacy, and ethical conduct. Addressing these challenges is paramount to realizing the full potential of machine learning for AI agents.
Looking Ahead: The Future of ML in AI Agents

In the future, as machine learning technology advances further, its implications on AI agent capabilities will accelerate exponentially. What the future will bring is not just smarter, and more autonomous, but also more emotionally intelligent, ethical, and collaborative AI agents. Here's what we can look forward to:
1. Human-Like Comprehension and Emotional Awareness
Within the next few years, AI agents driven by ML models will be able to identify and better understand human emotions. They will not only know what someone is saying but also how they feel when they say it. This emotional awareness will allow AI agents to respond more compassionately in fields such as mental health care, customer support, and education- more naturally and based on trust.
2. Multi-Agent Collaboration and Coordination
Future AI agents won't operate alone. Machine learning will allow them to collaborate and coordinate with other agents, exchange information, and solve problems together. For example, in logistics or healthcare systems, several agents may collaborate in real-time to allocate resources more efficiently or respond to acute crises.
AI agents of today frequently require domain-specific training. However, with advancements in transfer learning and general-purpose models, agents will seamlessly be able to use the knowledge of one domain in another. For instance, an AI used for customer care in retail will easily find its application in finance or health without much reprogramming, courtesy of ML's generalizability.
3. Real-Time Learning and Adaptation
The future will hold AI agents that can learn and evolve continuously in real-time. With the help of online learning techniques and adaptive ML models, agents can instantly incorporate new knowledge, self-correct errors, and adapt their strategies on the fly—without requiring periodic model updates.
As AI gains more trust, future machine-learning models will be more explainable. Users and developers will have the ability to see why a particular decision was made by an agent, avoiding bias and becoming more transparent. Additionally, ethical frameworks will be incorporated into AI agents so they can behave responsibly and make choices that reflect human values.
4. Hyper-Personalized User Experiences
With greater understanding based on behavioral information, next-generation AI agents will provide hyper-personalized service- modulating their tone, recommendations, and behavior for every user. From adaptive learning for education to goal-growing personal finance bots, machine learning in AI agents will make digital experiences reach an unprecedented degree of customization.
5. Cross-Language and Cross-Cultural Intelligence
As ML algorithms become better at processing natural language, AI agents will break down language barriers with greater ease. They will not only translate phrases but also pick up on cultural references, sarcasm, and tone, making truly global and inclusive communication a reality.
Conclusion
The incorporation of machine learning in AI agents is that which converts them from rule-abiding programs into adaptive, knowing systems that advance with time and data. The more that AI is being deeply embedded into various aspects of human life, the more the combined power of ML and AI agents is poised to propel the future wave of digital transformation.
At Softude, we’re at the forefront of this evolution- leveraging the power of machine learning in AI agents to deliver smarter customer experiences, intelligent automation, and scalable solutions tailored to unique business needs. Whether it’s building personalized virtual assistants, predictive systems, or autonomous decision-making platforms, our team is passionate about pushing the boundaries of what AI agents can do.
The possibilities are endless, and at Softude, we are proud to be building the future, one intelligent solution at a time.
Liked what you read?
Subscribe to our newsletter