10 Actionable AI Tips for Enhancing Your Business Intelligence Process
- SoftudeFebruary 25, 2025
- Last Modified onFebruary 25, 2025
Enhancing a business intelligence (BI) process is essential in the current data-centric world. Slow processes create inefficiencies, mistakes, and lost opportunities. Companies usually wrestle with data quality problems, tedious processes, and the failure to derive rich insights.

Such constraints restrict decision speed and accuracy. Incorporating Artificial Intelligence (AI) in BI processes can eliminate these issues. The following ten tips illustrate common challenges and provide step-by-step solutions to re-engineer your BI processes.
How AI Can Transform Your Business Intelligence Process
1. Automate Data Cleansing & Preparation
The Problem
Manual data cleansing is labor-intensive and error-prone. Data usually comes from various sources in different formats. Inconsistencies, duplicates, and missing values form unreliable datasets. These problems slow down the analysis and generate wrong insights.
How This Tip Can Help

Data cleansing (fixing or deleting erroneous records) is a building block of BI. If data is inconsistent, the analysis will be unreliable. AI tools scan big data to detect anomalies. They normalize formats, eliminate duplicates, and insert missing values when feasible. This automated process minimizes the chance of human error and speeds up data preparation. With clean data, follow-up analyses are more trustworthy, resulting in more precise business insights.
2. Utilize AI-Powered Data Analytics Tools
The Problem
Conventional analytics tools do not always effectively reveal faint patterns in large data sets. Most organizations are challenged when attempting to derive useful insights. Analysis can be hindered by the limitations of time and human miscalculations. This translates to lost opportunities for development and enhancement.
How This Tip Can Assist

AI-powered data analysis converts raw data into in-depth insights. Statistical models and ML are used in the technology to identify unseen patterns. Predictive modeling, for instance, can indicate upcoming trends even before they are self-evident. Automating analysis, these platforms ease the burden on analysts and deliver quicker, more accurate insights. This helps organizations respond instantly and make strategic decisions with in-depth data analysis.
3. Deploy Predictive Analytics
The Issue
Many organizations operate on historical data without anticipating future trends. Relying solely on past performance limits strategic planning. Reactive decision-making can lead to missed opportunities and increased risks. There is often a gap between current performance and future needs.
How This Tip Can Help
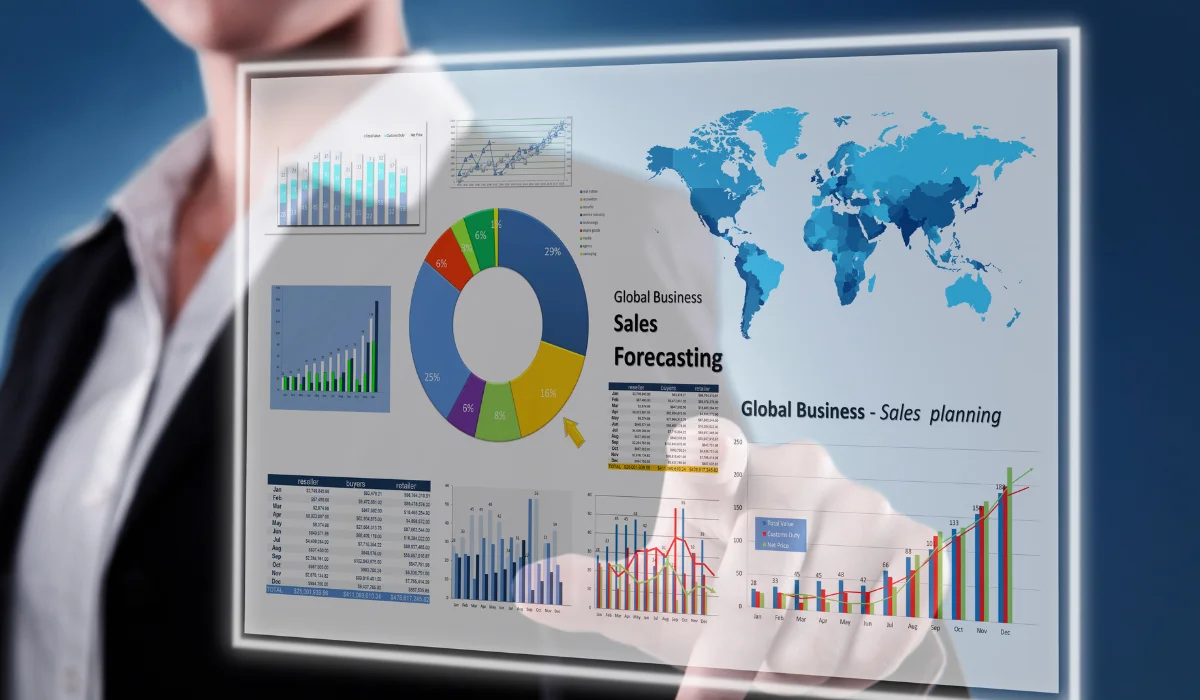
Predictive analytics leverages machine learning to apply statistical algorithms to historical data to create forecasts. These statistical models detect patterns in data and extrapolate into the future. By predicting future outcomes, organizations can make adjustments in advance. For example, demand forecasting can optimize supply chains and inventory management. The result is a more responsive organization that can act ahead of market changes.
4. Augment Data Visualization with AI
The Problem
Complicated data tends to look daunting when shown in unprocessed numerical form. Inefficiently designed visualizations can interfere with comprehension and delay decision-making. Most teams find it challenging to quickly comprehend data, which causes delays in recognizing key problems.
How This Tip Can Help

Visualization software powered by AI transforms intricate data sets into easy-to-understand, interactive charts. The software creates dynamic dashboards that automatically update in real-time. Users can drill down into individual data points, which makes the information more actionable and accessible.
5. Use Natural Language Processing (NLP) for Insight Generation
The Problem
Most business information is unstructured text: customer comments, social media updates, and internal reports. Textual data analysis is tedious and prone to missing important subtleties by hand. With no analysis, valuable insights go unrevealed.
How This Tip Can Help
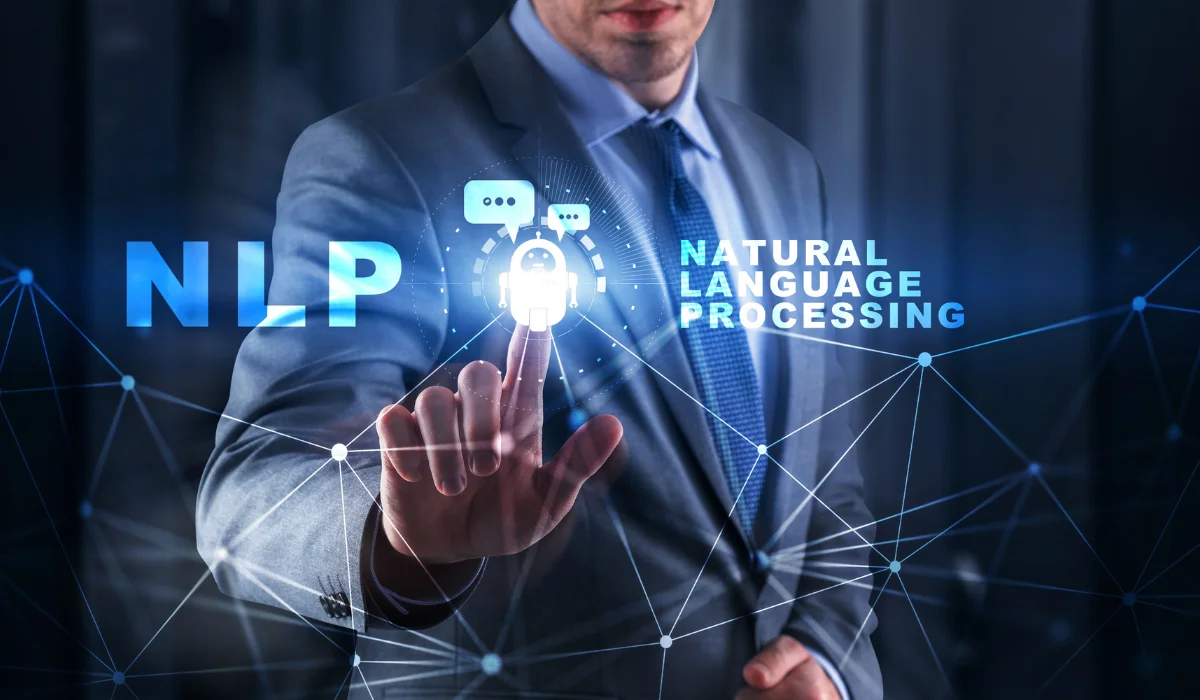
Natural Language Processing (NLP) is a machine-learning method that processes human language. It translates unstructured text into measurable data. NLP tools perform sentiment analysis, repeated patterns, and keyword extraction. It converts qualitative data into actionable insights.
6. Automate Reporting using Smart Dashboards
The Problem
Report creation is labor-intensive and, most of the time, results in stale information. Hand-written reports can be inaccurate and do not provide real-time data. Managers usually end up working with stale or incomplete information.
How This Tip Can Assist
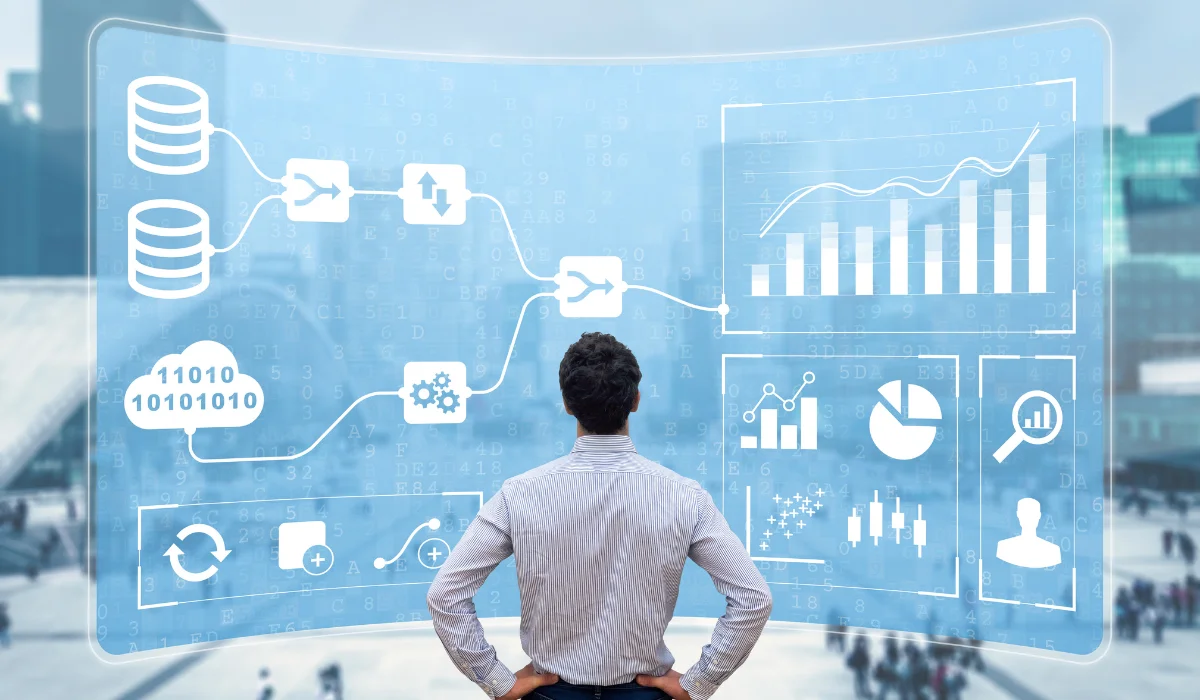
Smart dashboards streamline the task of assembling and refreshing reports. Smart dashboards pull in data from various sources and refresh on their own. They give real-time snapshots of key performance indicators (KPIs), making all the reports up-to-date and precise.
The application of AI ensures that these dashboards refresh regularly or when fresh data becomes available. This steady stream of up-to-date information eliminates the need for manual report running. Decision-makers get timely notices that allow instant and accurate reactions to developing problems.
7. Implement Real-Time Data Monitoring and Alerts
The Problem
In high-speed business settings, lag in issue detection can cause serious repercussions. Conventional monitoring techniques may fail to identify issues until they blow up. Companies tend to experience operational interruptions due to critical deviations going undetected.
How This Tip Can Assist

Real-time monitoring makes companies stay alert. AI systems continuously analyze data, comparing performance to historical patterns or established thresholds. Upon detection of abnormalities, the affected teams are notified. This advanced action reduces downtime and keeps small problems from snowballing into huge disruptions. The outcome is a more agile company that can respond quickly during emergencies.
8. Invest in an AI-powered BI Platform
The Problem
Having numerous disconnected tools causes data silos and inefficiency. Organizations end up with integration issues when different systems do not communicate well. This fragmentation slows the overall BI process and diminishes the return on technology investments.
How This Tip Can Assist
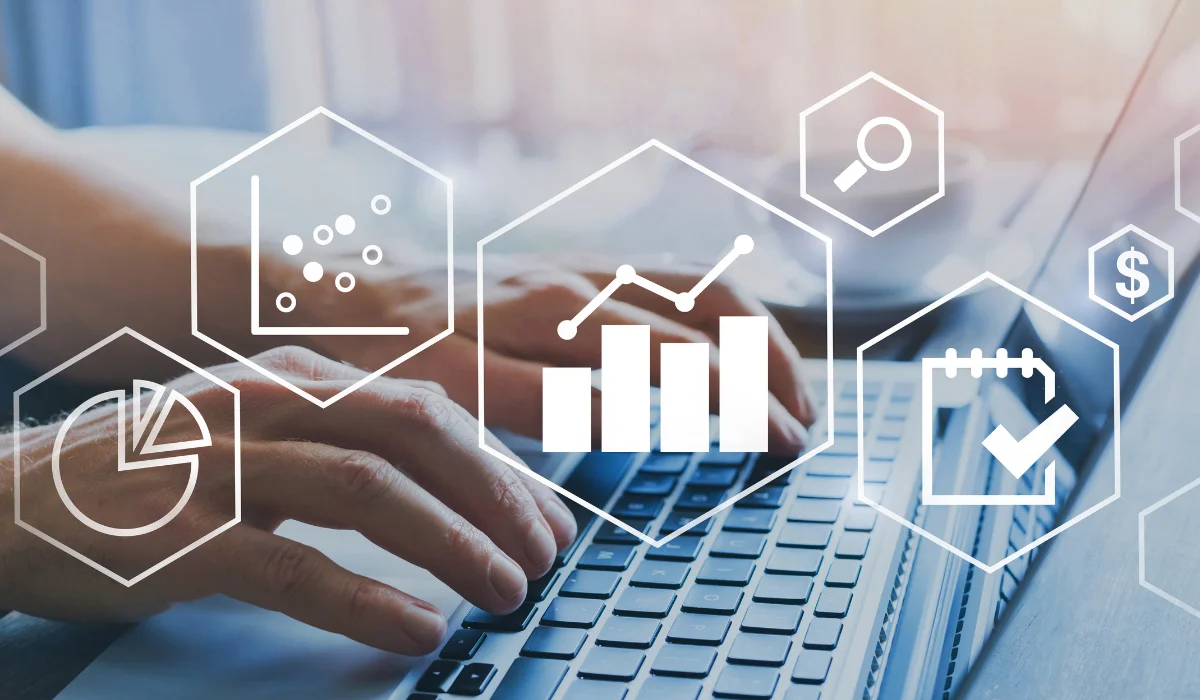
A centralized BI platform provides an end-to-end solution to handle data. By integrating numerous tools into one platform, companies remove the drag of data transition between different systems. AI optimizes the platform by streamlining processes and applying consistency to every function.
Taking a complete approach not only simplifies the flow but also brings a scalable answer that expands at the same pace as the growth of the company. The platform offers a higher return on investment with enhanced consistency of data and optimized operations.
9. Establish a Culture of Data Literacy & Innovation
The Issue
Even the finest technology will perform suboptimally if it lacks qualified users. Numerous organizations wrestle with inadequate data literacy, in which staff are unprepared to comprehend and respond to data insights. This deficiency prevents BI tools from being used efficiently and suppresses innovation.
How This Tip Can Help
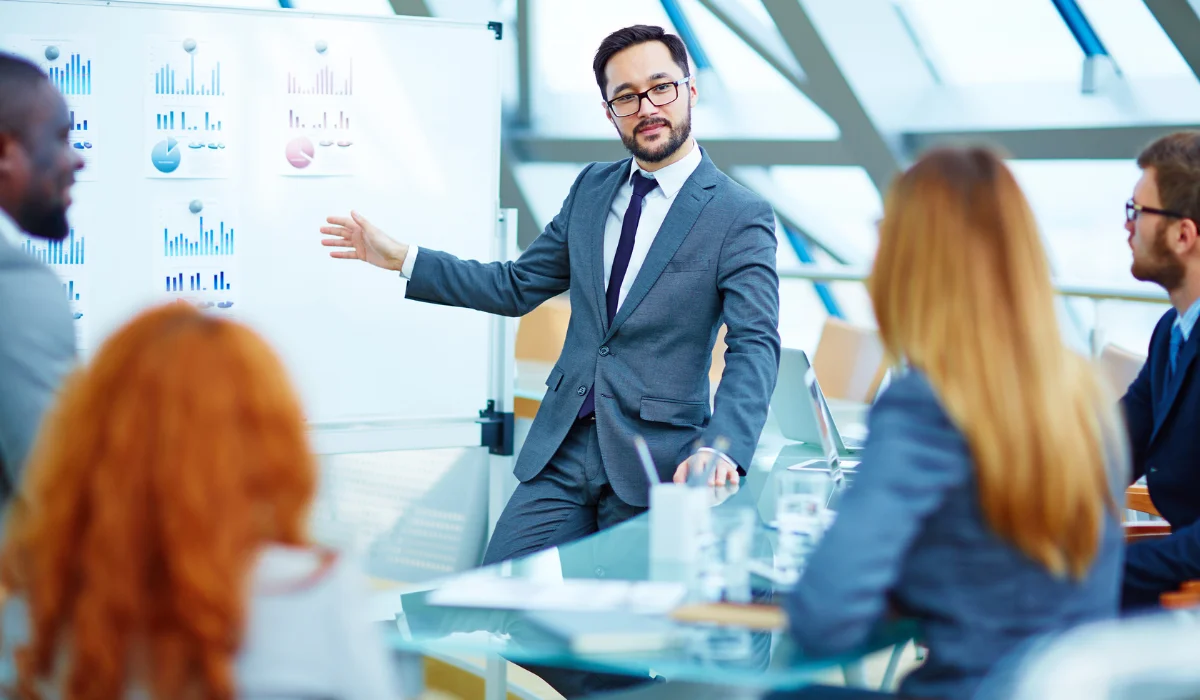
Data literacy is reading, working with, analyzing, and communicating data. Investing in training programs guarantees that every team member from executives to new hires possesses the skills to utilize BI tools effectively. Promoting collaboration and innovation creates a culture where data-driven decisions are the norm. This cultural transformation optimizes the advantages of AI-driven BI tools and fuels ongoing improvement and a competitive edge.
10. Always Optimize and Iterate Your BI Workflow
The Problem
Static BI workflows become obsolete in a rapidly changing business landscape. Without periodic reviews, inefficiencies and new issues can remain unresolved. Organizations stand to lose ground as new processes and technologies are developed.
How This Tip Can Assist

Optimization is a continuous process. Ongoing reviews of the BI workflow identify areas where bottlenecks and improvement opportunities exist. User feedback gathering and performance metric monitoring are key actions.
AI-powered analytics can detect trends in workflow efficiency, leading to changes required. This continuous improvement focus means that the BI system remains dynamic and efficient and can respond to changing business requirements.
Conclusion
Streamlining the BI workflow is crucial to converting data into actionable insights. These ten AI tips solve typical problems and give an elaborate solution. Automation, integration, and constant optimization are important in minimizing errors, saving time, and improving decision-making. These strategies form a strong and flexible foundation for BI implementation by resolving data quality, manual processes, and real-time insight requirements.
Organizations that adopt these practical recommendations can anticipate enhanced accuracy, accelerated reporting, and greater returns on their data efforts. The outcome is a streamlined, efficient process that fuels informed decision-making and enables long-term business success.
Liked what you read?
Subscribe to our newsletter