Difference Between AI and ML: How They Are Used in the Real World
- SoftudeDecember 20, 2024
- Last Modified onDecember 20, 2024
Artificial intelligence and machine learning are among the most transformational technologies of the twenty-first century. Although the two names are frequently used interchangeably, they are essentially different. Understanding the differences between AI and ML is critical for firms seeking a competitive advantage.
In this guide, we will look at the differences between artificial intelligence and machine learning, how they function, how they are used in many industries, and how they will shape the future of business and society.
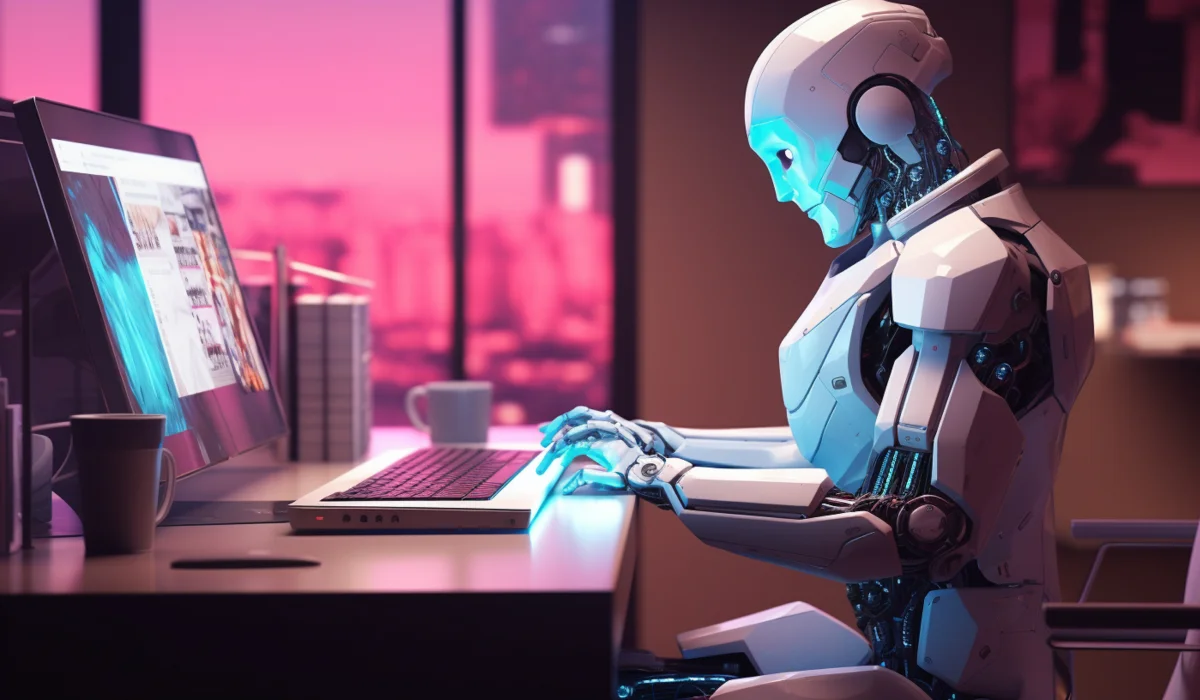
The Evolution of AI and Machine Learning
Artificial intelligence is in fact an offshoot that originated in the 1950s thanks to visionaries such as Alan Turing and John McCarthy the father of Artificial Intelligence. In the beginning, AI tried to imitate human intellect in a limited, rule-based environment. The initial approaches were coded with conventional rules and did not possess a learning ability or sensitivity to new datasets.
At some point, reliance on rules in AI systems transitioned to machine learning, a subfield, which enables machines to learn from examples with no need for programming improvements with time. This evolution resulted in the creation of more sophisticated systems capable of processing complex, unstructured data such as images, text, and speech.
What is Artificial Intelligence (AI)?
Artificial Intelligence refers to creating machines who can do tasks like humans. The systems are designed to do tasks that usually need human intelligence particularly problem solving, decision making and language processing.
AI can be categorized into two types:
Narrow AI (Weak AI): These systems are intended to fulfill a given function. But it’s not teaching them to generalize beyond that task, they’re trained to solve one particular problem.
General AI (Strong AI): This type of AI has the capability to handle any intellectual task that a human can perform. It remains largely theoretical, with no current systems achieving this level of capability.
Examples of AI
Heuristic DENDRAL, PXDES, and MYCIN are the AI that do not use Machine Learning. They come under the category of expert systems which are a sort of artificial intelligence that makes decisions based on a knowledge base and inference rules. Instead of learning from data, these systems handle problems using established logic.
What is Machine Learning (ML)?
Artificial Intelligence derives from Machine learning. This is a category of Artificial Intelligence that focuses on the use of data to train a machine in a way that the machine can improve on its performance. Unlike traditional programming, where explicit rules are defined by humans, ML algorithms learn themselves from patterns they find in data.
ML can be categorized into three types:
Supervised Learning: Model gets training on the labeled dataset that has both input features and corresponding output. It creates itself a mapping of the input to the right output using the provided labels.
Unsupervised Learning: In other words, this type of learning is about working with unlabeled data. The model analyzes the data and finds hidden patterns or structures within the data without any predefined labels or outputs.
Reinforcement Learning: If you are familiar with the term trial and error, this is definitely a type of learning from trial and error. It goes out in the world, interacts with an environment and learns from the results then selects the best choice in order to maximize reward.
What is an Example of Machine Learning?
An example of machine learning is the recommendation system used by Netflix. This system uses historical data about user preferences and behaviors to predict and recommend movies and TV shows to watch. And as the system has more data over time, their predictions get refined and the recommendations get better.
AI vs. ML: Key Differences
1. Scope
- Artificial intelligence (AI) refers to machines that do jobs often done by humans.
- Machine learning (ML) is a subset of artificial intelligence that allows machines to learn from data without explicit programming.
2. Functionality
- AI attempts to recreate or emulate thinking, perceiving or perceiving and deciding which are cognitive functions associated with a human being.
- ML is the procedure of designing models that can analyze or evaluate data in a way that allows them to enhance a particular performance.
3. Dependency
- AI does not always necessitate machine learning. Rule-based systems, for example, are AI applications that do not use machine learning.
- ML is fundamentally linked to AI because it is a methodology within the larger area of AI.
Difference Between Artificial Intelligence and Machine Learning Engineer
AI Engineer: An engineer in artificial intelligence develops technologies that are capable of performing human-like intelligence. They work on natural language processing, speech recognition, and cognitive computing.
ML Engineer: An ML engineer develops and optimizes algorithms to train machines on data. They are performed on ‘big’ data and make predictions and classification for data. It involves communication, analysis, and implementation with all stakeholders both in terms of IT and business related compliance and monitoring to enable the effective operation of AI and ML frameworks
Both positions are critical in the deployment of AI and ML solutions, but the primary distinction is in the scope of their job.
AI and ML Use Cases in the Real World
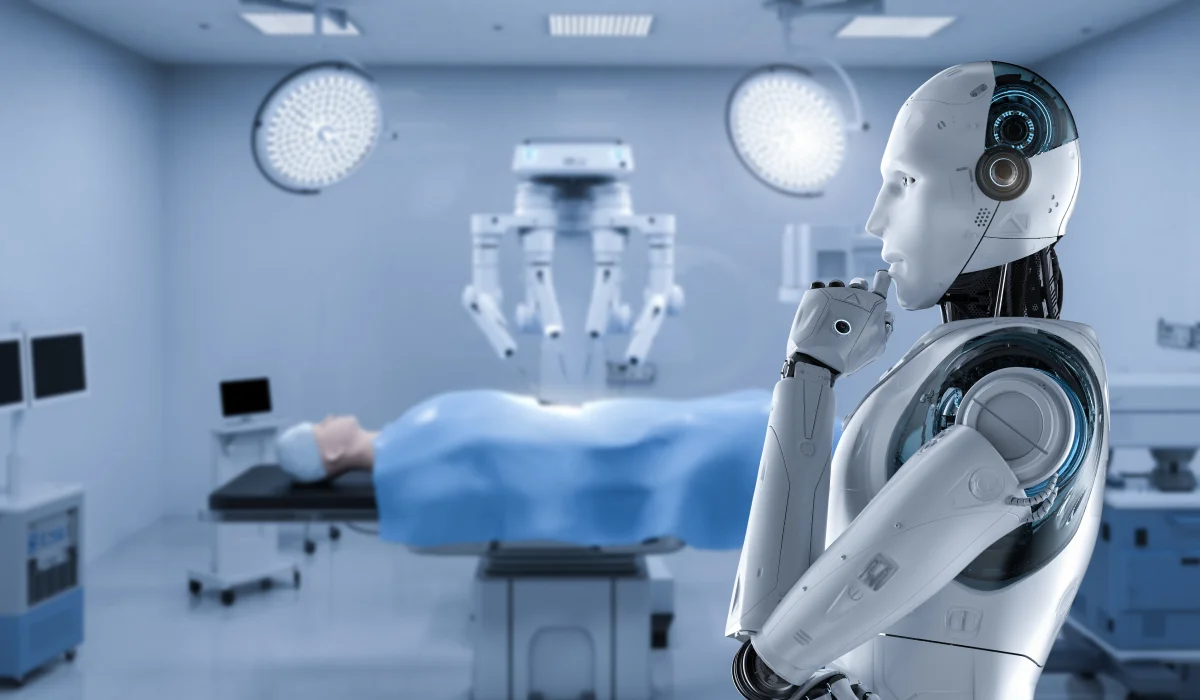
1. Healthcare
AI and ML are revolutionizing the perception of healthcare as it can make diagnoses standards better, the treatments personalized and enhance the flow of the hospital. AI systems are analyzing the medical images to anticipate an emergent disease or help identify a drug.
2. Retail
In the retail industry, AI and ML are powering personalized recommendation, smart inventory management and dynamic pricing through Artificial Intelligence. They are also aiding customer service by answering queries and processing transactions.
3. Finance
Fraud detection, automatic credit risk evaluation, risk management, and algorithmic trading are a few areas where AI and ML are popularly used.
4. Automotive
Personalized infotainment systems, predictive maintenance, intelligent route finder, etc are the most popular applications of AI and Machine Learning.
How AI and ML Can Help Businesses
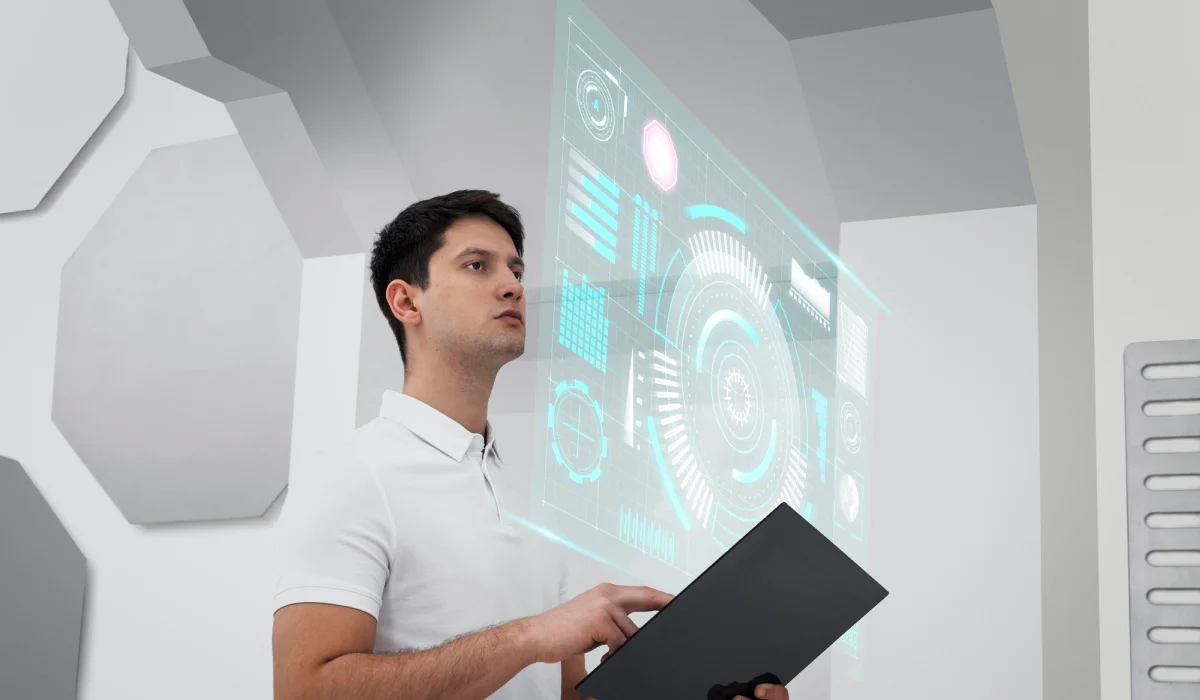
1. Data Driven Insights for Making Better Decisions
AI and ML work on data to guide you to make accurate decisions. By analyzing huge datasets these technologies analyze large datasets in an attempt to discover hidden trends and patterns so companies can more quickly react to changes in the market, changes in consumers behavior and changes in the industry.
2. Boosting Efficiency with Automation
AI-driven automation simplifies repetitive tasks, freeing employees to tackle more complex and creative work. Automating workflows is becoming industry standard in customer service, finance and retail companies to increase speed and accuracy.
3. Personalizing Customer Experiences
AI and ML allows businesses to know the customer's behavior and anticipate his future needs. For example, retail companies are using AI to create personalized products for customers.
4. Improving Product and Service Offerings
With machine learning, businesses can continuously improve their products and services. That’s where ML algorithms come in to analyze customer feedback and product performance to find out which areas can be improved and the business should keep an eye on competitors.
5. Predicting Future Trends
Machine learning models are helping businesses to see the future based on their current data. This predictive power pushes businesses to transition from a reactive to a proactive stage, where they are already prepared with a better plan to deal with the future.
6. Enhancing Security
AI and ML have a very important role helping security determine possible threats and vulnerabilities. In cybersecurity, AI helps in detecting unusual patterns in network traffic and prevent malicious attacks before they happen.
Challenges of AI and ML Adoption
While the potential of AI and ML is immense, businesses face several challenges in adopting these technologies:
Data Quality and Availability: Since you need to train the ML models you require high quality labeled data. However, the attainment of such data may be costly and time-consuming.
Ethical Concerns: Recently it was shown that AI systems can learn prejudices existing in the set data base and act in a prejudiced and unethical manner.
Cost: Because AI and ML-driven solutions can increase productivity by 30X to 100X, there is a significant tradeoff for the additional investment in infrastructure, extra human resources, and data that is needed.
The Future of AI and ML

AI and ML is a space filled with options regarding what the future may look like. As technology gets better each day, we can expect even more breakthroughs in areas such as:
Quantum Computing: What started off as a promising avenue to quantum-computing systems has become a race to see who develops the first genuine quantum computer that can solve the complex problems that are at the core of modern AI and ML.
AI for Sustainability: AI will be an important tool in overcoming the powers of climate change by automating energy usage, monitoring of ecosystems, and in the area of green technologies.
Human-AI Collaboration: With more collaboration between humans and AI systems, our work will involve more AI augmenting human capabilities rather than being a replacement.
FAQs
1. Is ChatGPT AI or ML?
ChatGPT is an AI built using ML techniques. It is based on GPT (Generative Pre-trained Transformer) models, which use machine learning techniques to process and generate human-like text based on input.
2. What Are the Basics of AI and ML?
AI focuses on enabling machines to simulate human intelligence whereas ML focuses on using algorithms and data to learn and improve performance.
3. Is ML the Same as AI?
No, ML is a subset of AI. While AI covers a wide range of intelligent systems, ML specifically focuses on those that learn from data.
Conclusion
It is Important to understand the connection between AI and Machine Learning in order to appreciate how both of them function and the best ways it may be used to assist in organizations. While AI is used to create systems that copy human behavior, ML focuses on enabling machines to learn from data. Both are disrupting and creating value streams that are changing the face of industries for most organizations.
If you are a business entrepreneur ready to deploy AI/ML technologies for your company, then let us help you. Softude provides AI and ML development services to help you lead the digital transformation curve.
Join us to define the role of technology solutions in the development of business and society together!
Liked what you read?
Subscribe to our newsletter